Genomics and radiomics: Tools to see the unseen to personalize radiation therapy
Images
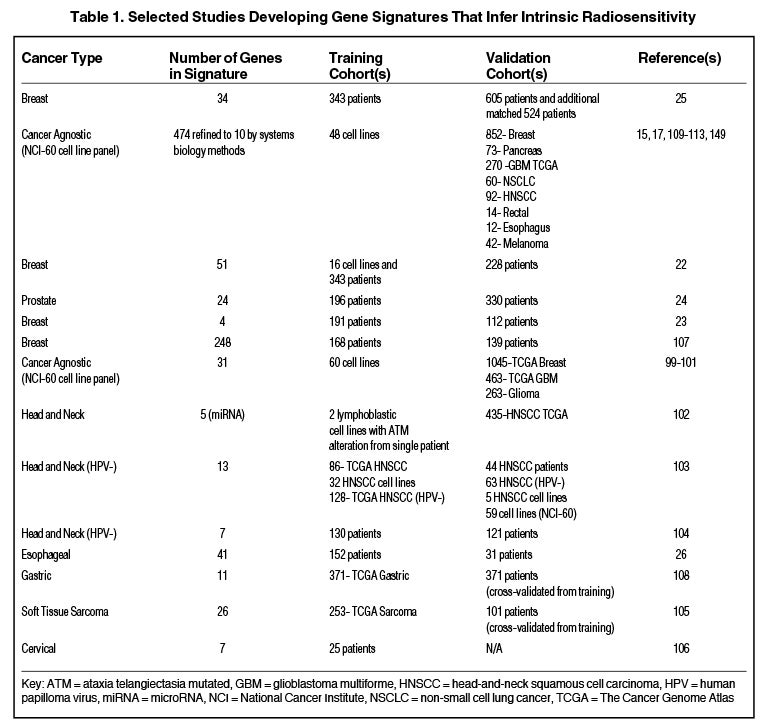
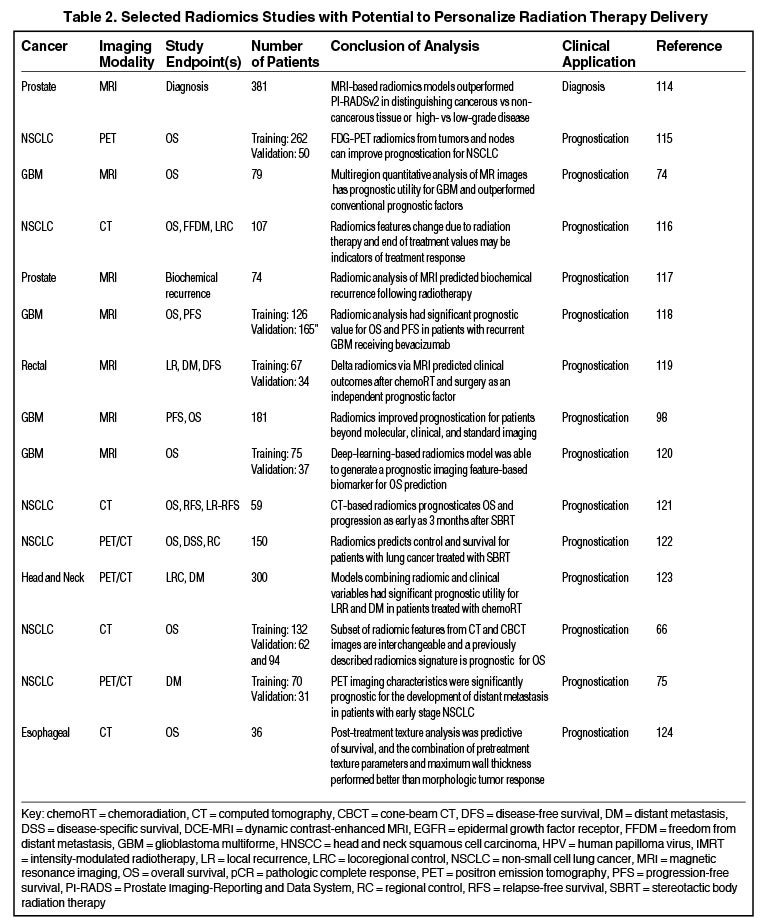
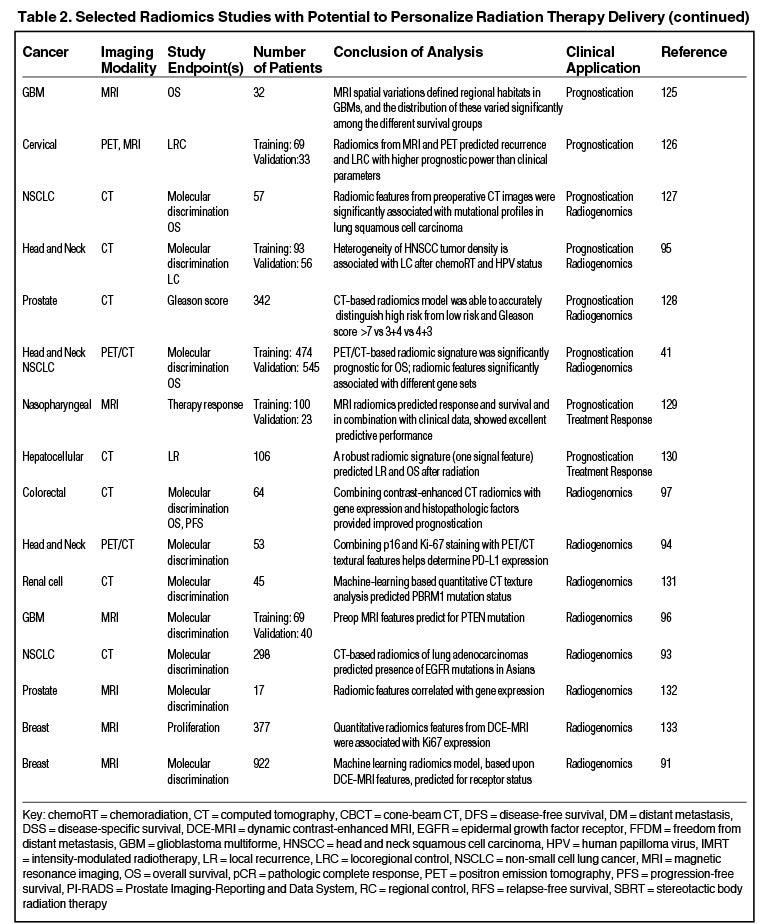
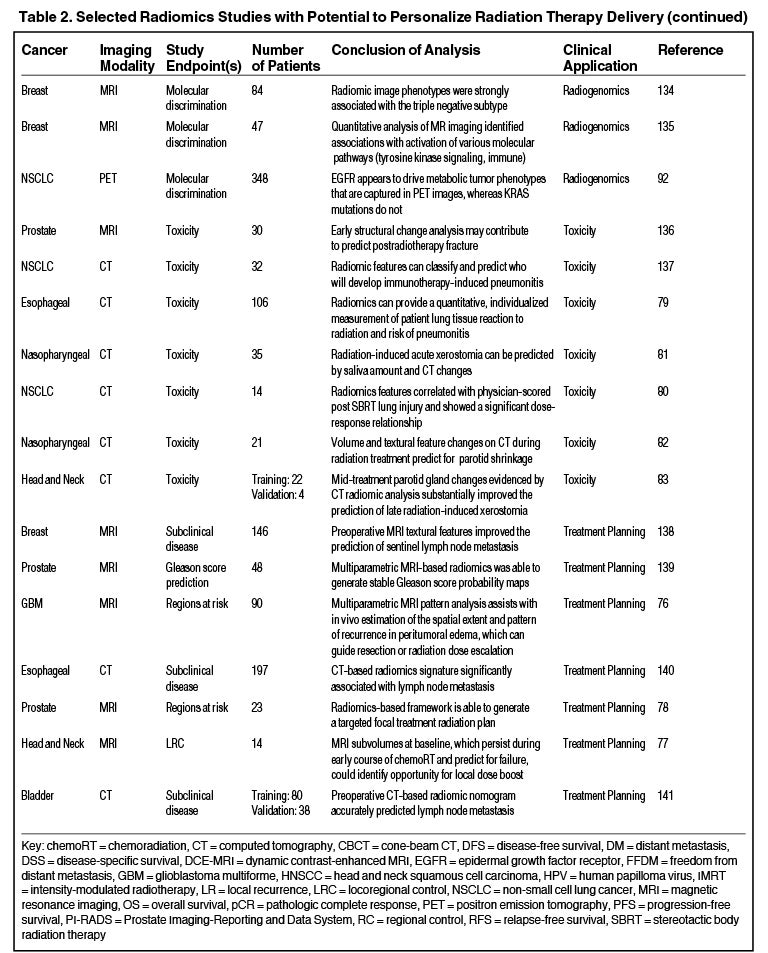
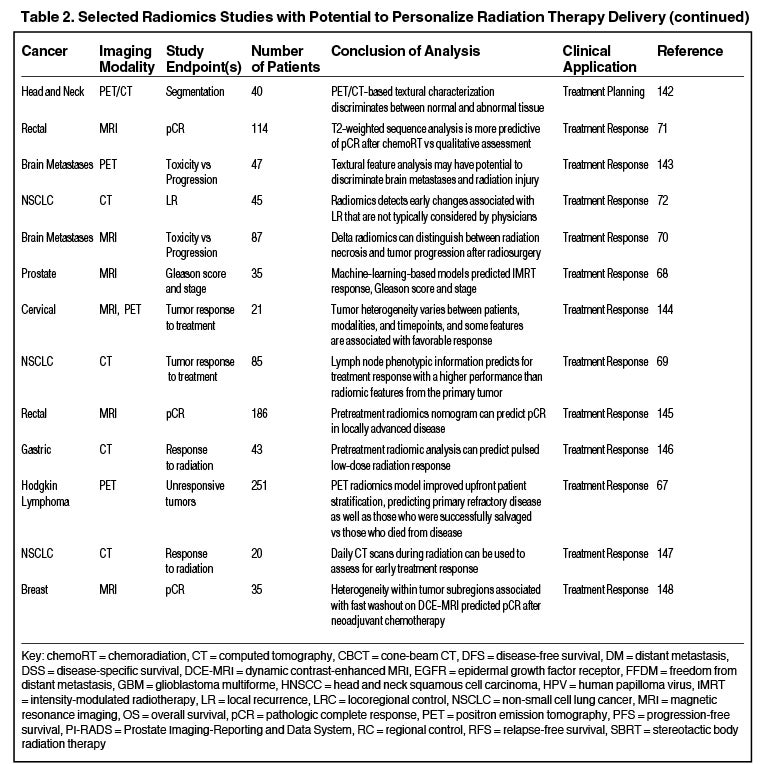
SA-CME credits are available for this article here.
Radiation therapy is a pillar of oncologic care for various solid malignancies in the curative and palliative settings. Technologic advancements spanning over a century have now provided opportunities for radiation to be delivered to the target of interest with high accuracy and precision. In this regard, implementation of 3-dimensional (3D) conformal radiation therapy and intensity-modulated radiation therapy (IMRT) coupled with daily image guidance have enhanced the achievable therapeutic ratio over a variety of dosing and fractionation schemes.1
Similar to medical oncology, radiation delivery has been guided by the fine balance of normal tissue toxicity and sustainability of durable tumor control. It is by these historical observations in which radiation dose has been chosen across a spectrum of malignancies and, to this day, remains the current dosing scheme for many cancers. Although it is generally accepted that tumors of the same stage, anatomic location and histology vary in their responses to radiation therapy, our field delivers treatment under a premise of established “clinical tolerance guidelines” rather than robust, tumor-specific, dose-response profiles.
In the last several decades, substantial advancements have been made in understanding the molecular catalog, metabolic networks and influence of the microenvironment on growth, spread and treatment response of various tumor types, yet employing these data in clinical decision-making has yet to inform the practice of radiation oncology. Furthermore, high-throughput analyses of clinically employed imaging modalities in radiation delivery has provided further opportunity to noninvasively categorize intrinsic tumor features and stratify patient outcomes. Further understanding of host and tumor differences with these interrogative approaches may provide the opportunity to precisely deliver radiation therapy beyond spatial and anatomic features, to one guided by intrinsic tumor biology.
Interrogation of Tumor Genomic Blueprints and Exploitation for Radiation Therapy
A major focus of personalized oncology has been the molecular characterization of tumors to identify unique druggable targets and generate higher order tumor classification methods to translate into clinical care.2 Numerous high-throughput “-omics” analyses, which encompass transcriptional, proteomic, methylation, metabolomic and sequencing data, have provided unprecedented insight into the underlying biology of various human tumors.3 These efforts have been largely performed within The Cancer Genome Atlas (TCGA) and International Cancer Genome Consortium (ICGC) programs, although several academic and commercial organizations now perform Clinical Laboratory Improvement Amendments (CLIA)-certified analyses of tumor tissue to complement these efforts.4
The beginnings of precision oncology began with prior laboratory work, which identified the first cancer-related gene mutation in HRAS several decades ago.5 Following this discovery, other somatic alterations have been identified in various tumors, which has formulated the notion that genetic alterations may be targeted in specific tumors. Notably, analysis of genomic data from patient tumors has provided opportunity to develop targeted agents against various proteins controlling kinases to epigenetic modulators.6 Additionally, the development of therapeutic monoclonal antibodies (mAb) has transformed oncologic care, most recently by modulating the host immune response to tumors.7 Many of these targeted agents have been employed in unselected metastatic cohorts, although tumor profiling has been able to separate responders from nonresponders based on intrinsic tumor features. Various trials employing molecular profiling have begun, though to date, no prospective trial has demonstrated a benefit to selecting targeted agents based on tumor genomic make-up.8
In contrast to targeted therapy selection, determination of the optimal radiation regimen may require a different approach. Ionizing radiation does not have a distinct “target,” but distributes its effect in the cell via a stochastic manner causing damage to DNA, organelles and cellular membranes.9 Additionally, at the tumor level, radioresponsiveness is influenced by other treatment parameters, including dose-volume relationships, total dose, fractionation pattern and type of radiation. In support of the latter, recent studies highlighted molecular differences in tumor cell radiosensitivity between dense and sparse ionizing rays10 and various dose per fraction regimens.11
Some of the first studies evaluating a molecular basis for radiation sensitivity were related to normal tissue toxicity in patients with alterations in ataxia telangiectasia mutated (ATM),12 which supported a DNA damage basis for intrinsic radiosensitivity. Numerous observations in single nucleotide polymorphism (SNP) analyses and experimental manipulations of DNA damage repair (DDR) modulators have supported this model, yet no clinically actionable genetic alteration has been validated.4 Interestingly, patients with rare genetic syndromes driven by compromised DDR pathways demonstrate a spectrum of responses, suggesting that a single alteration in core DDR machinery may not be a sole determinant of radioresponsiveness.13 Yard et al profiled more than 500 cell lines and identified interconnectedness between DDR protein alterations and genomic stability, which governed intrinsic radiosensitivity.14 This study underscores the polygenic trait of radiation sensitivity.
Attempts to model the polygenic nature of radiation sensitivity have continued to emerge in recent years. One of the first studies to address this question was by Eschrich et al, who identified a cancer-agnostic diverse gene network, which modeled the cellular survival following 2 Gy in 48 cancer cell lines.15 This network was reduced to 10 hub genes, from which a multigene expression signature was derived, termed the radiosensitivity index (RSI). The RSI has predicted for clinical outcomes in various patient cohorts treated with radiation,16 and recently Scott et al demonstrated that substitution of a tumor-specific RSI value for the alpha variable in the linear quadratic model derives an actionable tumor feature termed the genomically adjusted radiation dose (GARD),17 which can stratify clinical outcomes in patients treated with radiation.18,19
Others have hypothesized that tumor type-specific evaluation of radiation sensitivity may provide more robust classifiers compared to cancer-agnostic approaches, although some have suggested that despite heterogeneous sites of tumor origin, a common transcriptional program may regulate radiosensitivity.20,21 Table 1 is a nonexhaustive list of gene signatures developed to infer radiosensitivity. For instance, in breast cancer, Speers et al derived a transcriptional signature based on survival after 2 Gy in breast cancer cell lines and a patient cohort that predicted for local control in patients treated with radiation,22 and Tramm et al identified a 4-gene signature that predicted for postmastectomy radiation benefit.23 Similarly, the postoperative radiotherapy outcome score (PORTOS), a 24-gene signature in prostate cancer, has been validated as a predictive tool for assessing distant metastasis risk following postprostatectomy radiation.24
Combining gene signatures representing distinct biological processes may also improve the robustness of clinical classifiers. For example, Cui et al developed independent radiosensitivity and antigen processing/presentation signatures in breast cancer cohorts and found that integration of these signatures improved outcome stratification.25 Zhang et al also found that integrating a 31-gene signature with the RSI, both derived similarly from the NCI-60 cell line panel, improved predictive ability in esophageal cancer patients.26
Interestingly, many signatures proposed to delineate intrinsic radiosensitivity show little overlap, if any, with regard to gene sets. Is this due to a broadly conserved transcriptional program resulting from genotoxic stress or is there redundancy in the information of gene signatures? Despite publication of various gene signatures representing diverse biologic processes (eg, hypoxia, epithelial-mesenchymal transition, cell proliferation), prior studies have identified similarities in the predictive ability of diverse gene sets in a single dataset for similar clinical endpoints. For instance, Fan et al found a high concordance for nonoverlapping gene signatures in breast cancer, suggesting a common biologic underpinning.27
Few studies investigating relationships between gene signatures and clinical outcomes prove the specificity of the derived signature by testing against a negative control signature. A study by Venet et al found that gene signatures unrelated to cancer biology (ie, effect of postprandial laughter, skin fibroblast localization, social defeat in mouse brains) were associated with overall survival in a breast cohort and found that only 18 of 47 (40%) signatures from the literature had the ability to outperform random signatures of similar size.28
Functional redundancy of many gene signatures argues that robust statistical methods, including random permutation of genes selected to represent signature modules, are needed to avoid spurious associations with clinical outcomes.29 As the number of gene signatures continues to grow, it is important to interrogate the biology of individual genes composing the signature since sophisticated bioinformatics analyses can overcome real biologic differences and ultimately lead to no downstream utility.30
There are several important limitations to consider when implementing genomic-based strategies in clinical medicine. A major concern is the use of single-biopsy-site, tumor-profiling data to infer overall tumor biology. Tumors have significant spatial and temporal heterogeneity,31,32 often with opposing prognostic gene expression profiles or targetable mutations in different tumor regions. Although heterogeneity is evident, selection of many targeted therapies and clinically useful gene signatures is informed by single-region analyses,33 suggesting that the calculated signal in the readout may represent central biology in the tumor. Tumor profiling adds an additional level of complexity compared to signatures derived from cell cultures due to heterogeneous cell populations contributing to tumor composition. Aran et al found that noncancerous cell populations contribute to gene expression profiles and following adjustment for tumor purity, variation in differentially expressed genes and pathway enrichments were lost; this study emphasizes the need to correct for tumor purity.34
Another important feature to consider is the assumption that a snapshot of tumor biology derived from a single biopsy is representative of biology as treatment progresses. Myriad evidence demonstrates adaptive changes following exposure to various treatments.35,36 For example, radiation has been shown to induce alternative splicing,37 which has the potential to increase transcriptome diversity. Another example of adaptation is in prostate cancer cells exposed to enzalutamide, which results in differential expression of genes regulating inflammation and various metabolic processes.38 Thus, assuming an iso-effect response to each fraction of radiation may not provide a complete picture of the dynamicity in a responding tumor.39
Lastly, and of utmost importance, is the required external validation of derived signatures before adoption into clinical practice. Rigorous testing in prospective randomized clinical trials or prospectively collected retrospective analyses of previous phase III trials are required to demonstrate robustness of the signature outside of the training and nonprospectively collected validation cohorts. The utility of genomic-based approaches in radiation has lagged as none of the aforementioned signatures have withstood scrutiny of the protective regulatory barriers needed to safeguard patients from implementation in clinical decision-making.
Radiomics: A Noninvasive Means to Assess Tumor Biology
Routine medical imaging, including computed tomography (CT), magnetic resonance imaging (MRI) or positron emission tomography (PET), is paramount to the diagnosis, treatment and follow-up of cancer patients.40 Radiation oncologists approach data supplied by these anatomical and functional images differently than diagnostic radiologists, in that images are used to plan dose distributions that cover gross disease or regions at risk for spread. Although qualitative assessment by radiologists provides useful diagnostic information, each image contains a plethora of features that may be used for precise radiation delivery and treatment selection.
Radiomics refers to high-throughput extraction of quantitative image features from standard-of-care images, such as CT, MRI and PET followed by relation to biologic or clinical endpoints.41-43 This noninvasive process allows for the ability to describe tumor characteristics while accounting for spatial and temporal heterogeneity.44,45 Radiomics has the capacity to detect medical imaging phenotypes that are reflective of tumor features at the cellular level, with a prime example being 18-fluorodeoxyglucose PET (FDG-PET) representing glucose uptake.41 Also, data obtained from quantitative image analysis can identify novel tumor features that complement clinical or genetic characteristics, thus improving the understanding of tumor biology.43 Radiomics has potential to be a powerful tool to personalize clinical-decision algorithms, and novel methods continue to emerge for utilization in radiation therapy.
The workflow of radiomics involves several steps, including image acquisition, segmentation of the regions of interest (ROI), extraction of descriptive features, predictive modeling, and validation; each of these steps pose unique challenges described further below.42,45
Image Acquisition
The first step of radiomics involves acquiring standard-of-care images. Although lack of standardized imaging protocols across institutions does not significantly affect clinical utilization, these diverse protocols do impact extraction of quantitative features. Heterogeneous source data increase the probability for noise interference, calibration error and unfruitful analyses. For this reason, nonstandardized multi-institutional radiomics can pose major challenges. There have been recent attempts to address this issue through standardization of the imaging protocol, including the Quantitative Imaging Biomarkers Alliance (QIBA) and the Quantitative Imaging Network (QIN).46,47
Segmentation of Regions of Interest
The next step is segmentation of ROIs to determine which pixels/voxels within the image are to be analyzed. This step has been called the most challenging and contentious component of radiomics, and the segmentation process varies greatly across studies.42 The process can be conducted manually, which can introduce bias through user variability48 or can potentially be semi- or fully automated with newer approaches.49,50
Extraction of Descriptive Features
Radiomic features can be divided into spatial (static) and temporal (dynamic) features. Static features are derived from shape, volume, voxel intensity and texture, whereas dynamic features represent changes in kinetics with time-varying protocols.51 Semantic features, commonly used in radiology to qualitatively describe images (eg, spiculation, cavitation, necrosis), can be time-consuming to capture and do not provide more granular data for statistical modeling. Ongoing efforts with machine-learning methods strive to increase inter-reader agreement, lower variance, and augment more rapid data acquisition for semantic features.52 Agnostic features, which quantitatively describe heterogeneity within the ROI (eg, wavelets, textures, histogram characteristics) can provide statistical inter-relationships between voxels and reveal hidden patterns. These features can be calculated by various texture matrices (eg, gray-level co-occurrence, neighborhood gray tone difference matrix); for a more thorough description of feature calculation please see the recent article by Rizzo et al.53
The feature extraction process is variable across institutions with recent attempts to address this issue. The Image Biomarker Standardization Initiative (IBSI) is an international collaboration that works to standardize extraction of image biomarkers.54 Additionally, an open platform termed Computational Environment for Radiological Research (CERR) has been introduced to improve reproducibility, speed and clinical integration of radiomics research.55,56 Other open-source software to extract features includes RaCaT and LIFEx.57,58
Predictive Modeling and Validation
Following feature extraction, data interrogation via manual statistical analysis or machine learning, is conducted to test for relationships between features, clinical endpoints or other questions of interest in a training model. Model building from a small sample size relative to the number of features can result in reduced accuracy and risk of overfitting. This potentially may be obviated by predetermining subsets of features to analyze or removing highly correlated variables, yet there are notable statistical considerations when analyzing large datasets.59,60 Model validation, both internal and external, is a necessity for radiomics studies. Ideally, a successful model will perform similarly in training and validation cohorts. Beyond the scope of this article, Park et al provide a useful guide to assess model performance in radiomics.61 When constructing predictive models with multivariable analysis, guidelines from transparent reporting of a multivariable prediction model for individual prognosis or diagnosis (TRIPOD) can help maintain reproducibility and transparency.62
Clinical Applications
Radiomics can significantly impact clinical decision-making within oncology,42,45,63 including radiation.64 Due to the vast number of recent radiomics studies, indicated by a 50% increase in published studies between 2017 and 2018,65 we have highlighted a subset with potential to personalize radiation therapy (Table 2).
Prognostication
Numerous studies have shown the utility of radiomics in stratifying clinical outcomes. Aerts et al demonstrated a CT-based radiomics signature, which captured heterogeneity and had significant prognostic value in lung and head-and-neck cancer.41 Another recent study found that a subset of features extracted from planning CT and cone-beam CT (CBCT) scans are interchangeable, and CBCT-based signatures were prognostic for lung cancer survival.66
Treatment Response
Radiomics has the potential to predict radiation therapy response. A recent study demonstrated that a PET-based model developed with machine-learning improved prediction of primary refractory disease in Hodgkin lymphoma.67 Also, Abdollahi et al developed an MRI-based model that predicted radiation therapy response for prostate cancer patients.68 Another CT-based model based on lymph node phenotypic features was predictive of pathologic response after neoadjuvant chemoradiation in lung cancer and outperformed primary tumor feature sets.69 Zhang et al combined 5 MRI radiomic features to distinguish radiation necrosis from tumor progression in brain metastasis treated with the Gamma Knife (Stockholm, Sweden).70 Similarly, a T2-weighted MRI classifier outperformed qualitative assessment in diagnosing complete response in rectal cancer patients after neoadjuvant chemoradiation.71 Another CT-based signature outperformed physicians in identifying early changes associated with local recurrence after stereotactic ablative radiation therapy (SABR) for early stage lung cancer.72 Clearly, radiomics modeling in assessing treatment response is an area with future utility.
Treatment Planning
Another exciting area is the potential to improve radiation treatment planning and target selection. Quantitative image analysis allows for the identification of spatially explicit and distinct subregions, or habitats, of the tumor.73 These habitats may be the result of unique intratumor selection mechanisms and have been shown to have some clinical significance. For example, Cui et al demonstrated that MRI multiregion analysis outperformed conventional prognostic factors in glioblastoma74 and Wu et al showed subregions in PET and CT images were also more robust in predicting lung tumor control than commonly used prognostic parameters.75 Rathore et al developed an MRI signature, which provided in vivo estimation of spatial extent and pattern of tumor recurrence within peritumoral edema of glioblastoma; these high-risk areas may be optimal targets for dose intensification.76 Similarly, Wang et al utilized dynamic contrast-enhanced MRI to identify subvolumes of primary head-and-neck tumors at increased risk for local failure.77 Recently a multimodule framework called radiomics-based targeted radiation therapy planning (Rad-TRaP) was created, which employs MRI data, deformable image registration, and a feature-based dose plan.78
Toxicity
Radiomics also has the capacity to assess for and predict radiation-induced toxicity. Cunliffe et al identified changes in serial CT features that are associated with radiation dose and development of radiation pneumonitis.79 Another study identified CT-based texture features significantly correlated with dose and lung injury severity after SABR.80 Others have found that observed changes in radiomics-based measures (delta radiomics) over the course of radiation therapy predict for parotid gland shrinkage and xerostomia.81-83
Utility of Radiogenomics
There are 2 definitions of “radiogenomics” in the literature: 1) the study of genetic variation associated with radiation therapy response,84,85 and 2) the study of the relationship between gene expression patterns and imaging phenotypes;86,87 we refer to the latter.
One application of radiogenomics is to identify tumor imaging correlates of specific genomic attributes, which may provide a noninvasive alternative to biopsy.88,89 Multiple recent studies have shown the ability for MRI-based features to predict molecular subtypes and hormone receptor status in breast cancer.90,91 Other studies have demonstrated that radiomics can predict the presence of epidermal growth factor receptor (EGFR) mutations by PET features92 and CT features.93 Additionally, radiomics may be able to predict programmed death-ligand (PDL1) expression,94 human papilloma virus (HPV) status95 or a PTEN mutation.96
Others have shown that integrating radiomic and genomic data into a single model can improve prognostic power. For example, Badic et al used CT features and gene expression in colorectal cancer to improve patient stratification97 and Kickingereder et al found that an MRI signature combined with molecular and clinical data improved outcome prediction in glioblastoma.98
Pathways to Clinical Application
Genomic medicine has provided substantial insights into tumor biology and this has been exploited by medical oncologists in several facets of clinical practice and trial development.152 An advantage medical oncology has over radiation oncology in utilizing genomic information, is access to numerous biomarker panels with established FDA-approved targeted therapies. In contrast, commonly, radiation is an “add-on” modality in genomic-based trials, such as those with Oncotype Dx (TAILOR RT; NCT03488693), targeted therapies (NCT03667820), conventional chemotherapy (NCT03609216) or immunotherapies.154 Although not formally developed to assess radiation efficacy, several molecular classifiers are being employed in breast cancer to make decisions for treatment intensification or omission.153
Our institution is planning to initiate the first genomic-based prospective clinical trials to guide radiation therapy dose in early 2020. As part of this effort, RSI is being established in the CLIA molecular laboratory at Moffitt, which will allow us to use RSI and GARD in clinical trials. Our initial focus will be in head and neck cancer where we will use RSI/GARD to guide radiation dose de-escalation for HPV-positive head and neck cancer patients. A second trial in triple negative breast cancer will utilize the RSI/GARD model to decide whether patients should receive a boost to the tumor bed following whole-breast radiation.
Radiomics has the potential to significantly improve precision medicine in the diagnosis, prognostication, and treatment planning for cancer patients. However, the current literature is limited by its retrospective nature, as well as significant heterogeneity between studies. To improve the quality, standardization, and reproducibility of future studies, Lambin et al developed the radiomics quality score (RQS), a homogeneous evaluation criterion that assesses radiomics studies based on 16 key components.45,151 Vallieres et al emphasized the importance of designing high-quality, fully transparent, and accessible studies to improve the clinical translation of radiomics.150 Ongoing prospective clinical trials are investigating the utility of radiomics to inform clinical decision-making in the treatment of hepatocellular carcinoma (NCT03917017), prostate cancer (NCT03979573), and head-and-neck cancer (NCT03953976, NCT02666885). A trial in lung cancer plans to prospectively collect PET/CT data to predict response to immunotherapy (NCT04007068). However, further prospective validation, using the RQS as a guideline, is required to fully realize the potential of radiomics.
Conclusion
Big data analytics is rapidly progressing and demonstrates enormous potential to change the oncologic decision-making landscape. As improvements continue in bioinformatics, image analysis, statistical/machine learning models, and end-user experience with data interpretation, integration into the clinical workflow of a radiation oncologist is bound to occur soon. Genomics and radiomics provide an opportunity to increase the precision of radiation delivery in selection of dose and spatial delivery. Our field should openly embrace these tools and take the needed steps away from a “one-size-fits-all” philosophy.
References
- Beaton L, Bandula S, Gaze MN, Sharma RA. How rapid advances in imaging are defining the future of precision radiation oncology. Br J Cancer. 2019;120(8):779-790. doi: 10.1038/s41416-019-0412-y.
- Chin L, Andersen JN, Futreal PA. Cancer genomics: from discovery science to personalized medicine. Nat Med. 2011;17(3):297-303. doi: 10.1038/nm.2323.
- Chakraborty S, Hosen MI, Ahmed M, Shekhar HU. Onco-Multi-OMICS approach: a new frontier in cancer research. Biomed Res Int. 2018;9836256. doi: 10.1155/2018/9836256.
- Bergom C, West CM, Higginson DS, et al. The implications of genetic testing on radiotherapy decisions: a guide for radiation oncologists. Int J Radiat Oncol Biol Phys. 2019;S0360-3016(19)33530-33538. doi: 10.1016/j.ijrobp.2019.07.026.
- Tsuchida N, Murugan AK, Grieco M. Kirsten Ras* oncogene: significance of its discovery in human cancer research. Oncotarget. Jul 19 2016;7(29):46717-46733. doi: 10.18632/oncotarget.8773.
- Gatzka MV. Targeted tumor therapy remixed-an update on the use of small-molecule drugs in combination therapies. Cancers (Basel). 2018;10(6). doi: 10.3390/cancers10060155.
- Singh S, Kumar NK, Dwiwedi P, et al. Monoclonal antibodies: a review. Curr Clin Pharmacol. 2018;13(2):85-99. doi: 10.2174/1574884712666170809124728.
- Zimmer K, Kocher F, Spizzo G, Salem M, Gastl G, Seeber A. Treatment according to molecular profiling in relapsed/refractory cancer patients: a review focusing on latest profiling studies. Comput Struct Biotechnol J. 2019;17:447-453. doi: 10.1016/j.csbj.2019.03.012.
- Baskar R, Dai J, Wenlong N, Yeo R, Yeoh KW. Biological response of cancer cells to radiation treatment. Front Mol Biosci. 2014;1:24. doi: 10.3389/fmolb.2014.00024.
- Yard BD, Gopal P, Bannik K, Siemeister G, Hagemann UB, Abazeed ME. Cellular and genetic determinants of the sensitivity of cancer to alpha-particle irradiation. Cancer Res. 2019. doi: 10.1158/0008-5472.CAN-19-0859.
- Vanpouille-Box C, Alard A, Aryankalayil MJ, et al. DNA exonuclease Trex1 regulates radiotherapy-induced tumour immunogenicity. Nat Commun. 2017;8:15618. doi: 10.1038/ncomms15618.
- Taylor AM, Harnden DG, Arlett CF, et al. Ataxia telangiectasia: a human mutation with abnormal radiation sensitivity. Nature. 1975;258(5534):427-429. doi: 10.1038/258427a0.
- Kerns SL, Chuang KH, Hall W, et al. Radiation biology and oncology in the genomic era. Br J Radiol. 2018;91(1091):20170949. doi: 10.1259/bjr.20170949.
- Yard BD, Adams DJ, Chie EK, et al. A genetic basis for the variation in the vulnerability of cancer to DNA damage. Nat Commun. 2016;7:11428. doi: 10.1038/ncomms11428.
- Eschrich S, Zhang H, Zhao H, et al. Systems biology modeling of the radiation sensitivity network: a biomarker discovery platform. Int J Radiat Oncol Biol Phys. 2009;75(2):497-505. doi: 10.1016/j.ijrobp.2009.05.056.
- Oliver DE, Mohammadi H, Figura N, et al. Novel genomic-based strategies to personalize lymph node radiation therapy. Semin Radiat Oncol. 2019;29(2):111-125. doi: 10.1016/j.semradonc.2018.11.003.
- Scott JG, Berglund A, Schell MJ, et al. A genome-based model for adjusting radiotherapy dose (GARD): a retrospective, cohort-based study. Lancet Oncol. 2017;18(2):202-211. doi: 10.1016/S1470-2045(16)30648-9.
- Ahmed KA, Liveringhouse CL, Mills MN, et al. Utilizing the genomically adjusted radiation dose (GARD) to personalize adjuvant radiotherapy in triple negative breast cancer management. EBioMedicine. 2019;47:163-169. doi: 10.1016/j.ebiom.2019.08.019.
- Ahmed KA, Scott JG, Arrington JA, et al. Radiosensitivity of lung metastases by primary histology and implications for stereotactic body radiation therapy using the genomically adjusted radiation dose. J Thorac Oncol. 2018;13(8):1121-1127. doi: 10.1016/j.jtho.2018.04.027.
- Hall JS, Iype R, Senra J, et al. Investigation of radiosensitivity gene signatures in cancer cell lines. PLoS One. 2014;9(1):e86329. doi: 10.1371/journal.pone.0086329.
- Amundson SA, Do KT, Vinikoor LC, et al. Integrating global gene expression and radiation survival parameters across the 60 cell lines of the National Cancer Institute Anticancer Drug Screen. Cancer Res. 2008;68(2):415-424. doi: 10.1158/0008-5472.CAN-07-2120.
- Speers C, Zhao S, Liu M, Bartelink H, Pierce LJ, Feng FY. Development and validation of a novel radiosensitivity signature in human breast cancer. Clin Cancer Res. 2015;21(16):3667-3677. doi: 10.1158/1078-0432.CCR-14-2898.
- Tramm T, Mohammed H, Myhre S, et al. Development and validation of a gene profile predicting benefit of postmastectomy radiotherapy in patients with high-risk breast cancer: a study of gene expression in the DBCG82bc cohort. Clin Cancer Res. 2014;20(20):5272-5280. doi: 10.1158/1078-0432.CCR-14-0458.
- Zhao SG, Chang SL, Spratt DE, et al. Development and validation of a 24-gene predictor of response to postoperative radiotherapy in prostate cancer: a matched, retrospective analysis. Lancet Oncol. 2016;17(11):1612-1620. doi: 10.1016/S1470-2045(16)30491-0.
- Cui Y, Li B, Pollom EL, Horst KC, Li R. Integrating radiosensitivity and immune gene signatures for predicting benefit of radiotherapy in breast cancer. Clin Cancer Res. 2018;24(19):4754-4762. doi: 10.1158/1078-0432.CCR-18-0825.
- Zhang Q, Bing Z, Tian J, et al. Integrating radiosensitive genes improves prediction of radiosensitivity or radioresistance in patients with oesophageal cancer. Oncol Lett. 2019;17(6):5377-5388. doi: 10.3892/ol.2019.10240.
- Fan C, Oh DS, Wessels L, et al. Concordance among gene-expression-based predictors for breast cancer. N Engl J Med. 2006;355(6):560-569. doi: 10.1056/NEJMoa052933.
- Venet D, Dumont JE, Detours V. Most random gene expression signatures are significantly associated with breast cancer outcome. PLoS Comput Biol. 2011;7(10):e1002240. doi: 10.1371/journal.pcbi.1002240.
- Cantini L, Calzone L, Martignetti L, et al. Classification of gene signatures for their information value and functional redundancy. NPJ Syst Biol Appl. 2018;4:2. doi: 10.1038/s41540-017-0038-8.
- Chibon F. Cancer gene expression signatures - the rise and fall? Eur J Cancer. 2013;49(8):2000-2009. doi: 10.1016/j.ejca.2013.02.021.
- Hinohara K, Polyak K. Intratumoral Heterogeneity: More than just mutations. Trends Cell Biol. 2019;29(7):569-579. doi: 10.1016/j.tcb.2019.03.003.
- Gerlinger M, Rowan AJ, Horswell S, et al. Intratumor heterogeneity and branched evolution revealed by multiregion sequencing. N Engl J Med. 2012;366(10):883-892. doi: 10.1056/NEJMoa1113205.
- Narrandes S, Xu W. Gene expression detection assay for cancer clinical use. J Cancer. 2018;9(13):2249-2265. doi: 10.7150/jca.24744.
- Aran D, Sirota M, Butte AJ. Systematic pan-cancer analysis of tumour purity. Nat Commun. 2015;6:8971. doi: 10.1038/ncomms9971.
- Derbal Y. The adaptive complexity of cancer. Biomed Res Int. 2018;2018:5837235. doi: 10.1155/2018/5837235.
- Sharma P, Hu-Lieskovan S, Wargo JA, Ribas A. Primary, adaptive, and acquired resistance to cancer immunotherapy. Cell. 2017;168(4):707-723. doi: 10.1016/j.cell.2017.01.017.
- Wahba A, Ryan MC, Shankavaram UT, Camphausen K, Tofilon PJ. Radiation-induced alternative transcripts as detected in total and polysome-bound mRNA. Oncotarget. 2018;9(1):691-705. doi: 10.18632/oncotarget.21672.
- Ghashghaei M, Niazi TM, Aguilar-Mahecha A, et al. Identification of a radiosensitivity molecular signature induced by enzalutamide in hormone-sensitive and hormone-resistant prostate cancer cells. Sci Rep. 2019;9(1):8838. doi: 10.1038/s41598-019-44991-w.
- Blyth BJ. The paradox of adaptive responses and iso-effect per fraction. Int J Radiat Biol. 2018;94(8): 737-742. doi: 10.1080/09553002.2017.1398435.
- Yankeelov TE, Mankoff DA, Schwartz LH, et al. Quantitative imaging in cancer clinical trials. Clin Cancer Res. 2016;22(2):284-290. doi: 10.1158/1078-0432.CCR-14-3336.
- Aerts HJ, Velazquez ER, Leijenaar RT, et al. Decoding tumour phenotype by noninvasive imaging using a quantitative radiomics approach. Nat Commun. 2014;5:4006. doi: 10.1038/ncomms5006.
- Gillies RJ, Kinahan PE, Hricak H. Radiomics: images are more than pictures, they are data. Radiology. 2016;278(2):563-577. doi: 10.1148/radiol.2015151169.
- Lambin P, Rios-Velazquez E, Leijenaar R, et al. Radiomics: extracting more information from medical images using advanced feature analysis. Eur J Cancer. 2012;48(4):441-446. doi: 10.1016/j.ejca.2011.11.036.
- Kim JY, Gatenby RA. Quantitative clinical imaging methods for monitoring intratumoral evolution. Methods Mol Biol. 2017;1513:61-81. doi: 10.1007/978-1-4939-6539-7_6.
- Lambin P, Leijenaar RTH, Deist TM, et al. Radiomics: the bridge between medical imaging and personalized medicine. Nat Rev Clin Oncol. 2017;14(12):749-762. doi: 10.1038/nrclinonc.2017.141.
- Buckler AJ, Bresolin L, Dunnick NR, Sullivan DC, Group. A collaborative enterprise for multi-stakeholder participation in the advancement of quantitative imaging. Radiology. 2011;258(3):906-914. doi: 10.1148/radiol.10100799.
- Kalpathy-Cramer J, Freymann JB, Kirby JS, Kinahan PE, Prior FW. Quantitative Imaging Network: data sharing and competitive AlgorithmValidation Leveraging The Cancer Imaging Archive. Transl Oncol. 2014;7(1):147-152. doi: 10.1593/tlo.13862.
- Balagurunathan Y, Kumar V, Gu Y, et al. Test-retest reproducibility analysis of lung CT image features. J Digit Imaging. 2014;27(6):805-823. doi: 10.1007/s10278-014-9716-x.
- Zhou W, Xie Y. Interactive contour delineation and refinement in treatment planning of image-guided radiation therapy. J Appl Clin Med Phys. 2014;15(1):4499. doi: 10.1120/jacmp.v15i1.4499.
- Whitfield GA, Price P, Price GJ, Moore CJ. Automated delineation of radiotherapy volumes: are we going in the right direction? Br J Radiol. 2013;86(1021):20110718. doi: 10.1259/bjr.20110718.
- Tseng HH, Wei L, Cui S, Luo Y, Ten Haken RK, El Naqa I. Machine learning and imaging informatics in oncology. Oncology. 2018:1-19. doi: 10.1159/000493575.
- El Naqa I, Ruan D, Valdes G, et al. Machine learning and modeling: Data, validation, communication challenges. Med Phys. 2018;45(10):e834-e840. doi: 10.1002/mp.12811.
- Rizzo S, Botta F, Raimondi S, et al. Radiomics: the facts and the challenges of image analysis. Eur Radiol Exp. 2018;2(1):36. doi: 10.1186/s41747-018-0068-z.
- Zwanenburg A, Leger S, Vallieres M., Lock S. Image biomarker standardisation initiative. 2016. https://arxiv.org/abs/1612.07003. Accessed September 10, 2019.
- Apte AP, Iyer A, Crispin-Ortuzar M, et al. Technical note: extension of CERR for computational radiomics: a comprehensive MATLAB platform for reproducible radiomics research. Med Phys. Jun 13 2018. doi: 10.1002/mp.13046.
- Deasy JO, Blanco AI, Clark VH. CERR: a computational environment for radiotherapy research. Med Phys. May 2003;30(5):979-985. doi: 10.1118/1.1568978.
- Nioche C, Orlhac F, Boughdad S, et al. LIFEx: A freeware for radiomic feature calculation in multimodality imaging to accelerate advances in the characterization of tumor heterogeneity. Cancer Res. 2018;78(16):4786-4789. doi: 10.1158/0008-5472.CAN-18-0125.
- Pfaehler E, Zwanenburg A, de Jong JR, Boellaard R. RaCaT: An open source and easy to use radiomics calculator tool. PLoS One. 2019;14(2):e0212223. doi: 10.1371/journal.pone.0212223.
- Chalkidou A, O’Doherty MJ, Marsden PK. False discovery rates in PET and CT studies with texture features: a systematic review. PLoS One. 2015;10(5):e0124165. doi: 10.1371/journal.pone.0124165.
- Leijenaar RT, Nalbantov G, Carvalho S, et al. The effect of SUV discretization in quantitative FDG-PET Radiomics: the need for standardized methodology in tumor texture analysis. Sci Rep. 2015;5:11075. doi: 10.1038/srep11075.
- Park SH, Han K. Methodologic guide for evaluating clinical performance and effect of artificial intelligence technology for medical diagnosis and prediction. Radiology. 2018;286(3):800-809. doi: 10.1148/radiol.2017171920.
- Collins GS, Reitsma JB, Altman DG, Moons KG. Transparent reporting of a multivariable prediction model for individual prognosis or diagnosis (TRIPOD): the TRIPOD statement. BMJ. 2015;350:g7594. doi: 10.1136/bmj.g7594.
- Yip SS, Aerts HJ. Applications and limitations of radiomics. Phys Med Biol. Jul 7 2016;61(13):R150-166. doi: 10.1088/0031-9155/61/13/R150.
- Gardin I, Gregoire V, Gibon D, et al. Radiomics: principles and radiotherapy applications. Crit Rev Oncol Hematol. 2019;138:44-50. doi: 10.1016/j.critrevonc.2019.03.015.
- Sollini M, Antunovic L, Chiti A, Kirienko M. Towards clinical application of image mining: a systematic review on artificial intelligence and radiomics. Eur J Nucl Med Mol Imaging. 2019. doi: 10.1007/s00259-019-04372-x.
- van Timmeren JE, Leijenaar RTH, van Elmpt W, et al. Survival prediction of non-small cell lung cancer patients using radiomics analyses of cone-beam CT images. Radiother Oncol. 2017;123(3):363-369. doi: 10.1016/j.radonc.2017.04.016.
- Milgrom SA, Elhalawani H, Lee J, et al. A PET radiomics model to predict refractory mediastinal Hodgkin lymphoma. Sci Rep. 2019;9(1):1322. doi: 10.1038/s41598-018-37197-z.
- Abdollahi H, Mofid B, Shiri I, et al. Machine learning-based radiomic models to predict intensity-modulated radiation therapy response, Gleason score and stage in prostate cancer. Radiol Med. 2019;124(6):555-567. doi: 10.1007/s11547-018-0966-4.
- Coroller TP, Agrawal V, Huynh E, et al. Radiomic-based pathological response prediction from primary tumors and lymph nodes in NSCLC. J Thorac Oncol. 2017;12(3):467-476. doi: 10.1016/j.jtho.2016.11.2226.
- Zhang Z, Yang J, Ho A, et al. A predictive model for distinguishing radiation necrosis from tumour progression after Gamma Knife radiosurgery based on radiomic features from MR images. Eur Radiol. 2018;28(6):2255-2263. doi: 10.1007/s00330-017-5154-8.
- Horvat N, Veeraraghavan H, Khan M, et al. MR imaging of rectal cancer: radiomics analysis to assess treatment response after neoadjuvant therapy. Radiology. 2018;287(3):833-843. doi: 10.1148/radiol.2018172300.
- Mattonen SA, Palma DA, Johnson C, et al. Detection of local cancer recurrence after stereotactic ablative radiation therapy for lung cancer: physician performance versus radiomic assessment. Int J Radiat Oncol Biol Phys. 2016;94(5):1121-1128. doi: 10.1016/j.ijrobp.2015.12.369.
- Gatenby RA, Grove O, Gillies RJ. Quantitative imaging in cancer evolution and ecology. Radiology. 2013;269(1):8-15. doi: 10.1148/radiol.13122697.
- Cui Y, Tha KK, Terasaka S, et al. Prognostic imaging biomarkers in glioblastoma: development and independent validation on the basis of multiregion and quantitative analysis of MR images. Radiology. 2016;278(2):546-553. doi: 10.1148/radiol.2015150358.
- Wu J, Gensheimer MF, Dong X, et al. Robust intratumor partitioning to identify high-risk subregions in lung cancer: a pilot Study. Int J Radiat Oncol Biol Phys. 2016;95(5):1504-1512. doi: 10.1016/j.ijrobp.2016.03.018.
- Rathore S, Akbari H, Doshi J, et al. Radiomic signature of infiltration in peritumoral edema predicts subsequent recurrence in glioblastoma: implications for personalized radiotherapy planning. J Med Imaging (Bellingham). 2018;5(2):021219. doi: 10.1117/1.JMI.5.2.021219.
- Wang P, Popovtzer A, Eisbruch A, Cao Y. An approach to identify, from DCE MRI, significant subvolumes of tumors related to outcomes in advanced head-and-neck cancer. Med Phys. 2012;39(8):5277-5285. doi: 10.1118/1. 4737022.
- Shiradkar R, Podder TK, Algohary A, Viswanath S, Ellis RJ, Madabhushi A. Radiomics based targeted radiotherapy planning (Rad-TRaP): a computational framework for prostate cancer treatment planning with MRI. Radiat Oncol. 2016;11(1):148. doi: 10.1186/s13014-016-0718-3.
- Cunliffe A, Armato SG, 3rd, Castillo R, Pham N, Guerrero T, Al-Hallaq HA. Lung texture in serial thoracic computed tomography scans: correlation of radiomics-based features with radiation therapy dose and radiation pneumonitis development. Int J Radiat Oncol Biol Phys. 2015;91(5):1048-1056. doi: 10.1016/j.ijrobp.2014.11.030.
- Moran A, Daly ME, Yip SSF, Yamamoto T. Radiomics-based assessment of radiation-induced lung injury after stereotactic body radiotherapy. Clin Lung Cancer. 2017;18(6):e425-e431. doi: 10.1016/j.cllc.2017.05.014.
- Liu Y, Shi H, Huang S, et al. Early prediction of acute xerostomia during radiation therapy for nasopharyngeal cancer based on delta radiomics from CT images. Quant Imaging Med Surg. 2019;9(7):1288-1302. doi: 10.21037/qims.2019.07.08.
- Scalco E, Fiorino C, Cattaneo GM, Sanguineti G, Rizzo G. Texture analysis for the assessment of structural changes in parotid glands induced by radiotherapy. Radiother Oncol. 2013;109(3):384-387. doi: 10.1016/j.radonc.2013.09.019.
- van Dijk LV, Langendijk JA, Zhai TT, et al. Delta-radiomics features during radiotherapy improve the prediction of late xerostomia. Sci Rep. 2019;9(1):12483. doi: 10.1038/s41598-019-48184-3.
- Brothwell MRS, West CM, Dunning AM, Burnet NG, Barnett GC. Radiogenomics in the era of advanced radiotherapy. Clin Oncol (R Coll Radiol). 2019;31(5):319-325. doi: 10.1016/j.clon.2019.02.006.
- Kerns SL, West CM, Andreassen CN, et al. Radiogenomics: the search for genetic predictors of radiotherapy response. Future Oncol. 2014;10(15):2391-2406. doi: 10.2217/fon.14.173.
- Mazurowski MA. Radiogenomics: what it is and why it is important. J Am Coll Radiol. 2015;12(8):862-866. doi: 10.1016/j.jacr.2015.04.019.
- Rutman AM, Kuo MD. Radiogenomics: creating a link between molecular diagnostics and diagnostic imaging. Eur J Radiol. 2009;70(2):232-241. doi: 10.1016/j.ejrad.2009.01.050.
- Pinker K, Shitano F, Sala E, et al. Background, current role, and potential applications of radiogenomics. J Magn Reson Imaging. 2018;47(3):604-620. doi: 10.1002/jmri.25870.
- Chen WS, Townsend JP, Yu JB. Radiation-specific clinical data should be included in existing large-scale genomic datasets. Int J Radiat Oncol Biol Phys. 2017;98(1):8-10. doi: 10.1016/j.ijrobp.2017.01.023.
- Pinker K, Chin J, Melsaether AN, Morris EA, Moy L. Precision medicine and radiogenomics in breast cancer: new approaches toward diagnosis and treatment. Radiology. 2018;287(3):732-747. doi: 10.1148/radiol.2018172171.
- Saha A, Harowicz MR, Grimm LJ, et al. A machine learning approach to radiogenomics of breast cancer: a study of 922 subjects and 529 DCE-MRI features. Br J Cancer. Aug 2018;119(4):508-516. doi: 10.1038/s41416-018-0185-8.
- Yip SS, Kim J, Coroller TP, et al. Associations between somatic mutations and metabolic imaging phenotypes in non-small cell lung cancer. J Nucl Med. 2017;58(4):569-576. doi: 10.2967/jnumed.116.181826.
- Liu Y, Kim J, Balagurunathan Y, et al. Radiomic features are associated with EGFR mutation status in lung adenocarcinomas. Clin Lung Cancer. 2016;17(5):441-448 e446. doi: 10.1016/j.cllc.2016.02.001.
- Chen RY, Lin YC, Shen WC, et al. Associations of tumor PD-1 ligands, immunohistochemical studies, and textural features in (18)F-FDG PET in squamous cell carcinoma of the head and neck. Sci Rep. 2018;8(1):105. doi: 10.1038/s41598-017-18489-2.
- Bogowicz M, Riesterer O, Ikenberg K, et al. Computed tomography radiomics predicts HPV status and local tumor control after definitive radiochemotherapy in head and neck squamous cell carcinoma. Int J Radiat Oncol Biol Phys. 2017;99(4):921-928. doi: 10.1016/j.ijrobp.2017.06.002.
- Li Y, Liang Y, Sun Z, et al. Radiogenomic analysis of PTEN mutation in glioblastoma using preoperative multi-parametric magnetic resonance imaging. Neuroradiology. 2019. doi: 10.1007/s00234-019-02244-7.
- Badic B, Hatt M, Durand S, et al. Radiogenomics-based cancer prognosis in colorectal cancer. Sci Rep. 2019;9(1):9743. doi: 10.1038/s41598-019-46286-6.
- Kickingereder P, Neuberger U, Bonekamp D, et al. Radiomic subtyping improves disease stratification beyond key molecular, clinical, and standard imaging characteristics in patients with glioblastoma. Neuro Oncol. 2018;20(6):848-857. doi: 10.1093/neuonc/nox188.
- Kim HS, Kim SC, Kim SJ, et al. Identification of a radiosensitivity signature using integrative metaanalysis of published microarray data for NCI-60 cancer cells. BMC Genomics. 2012;13:348. doi: 10.1186/1471-2164-13-348.
- Jang BS, Kim IA. A radiosensitivity gene signature and PD-L1 status predict clinical outcome of patients with invasive breast carcinoma in The Cancer Genome Atlas (TCGA) dataset. Radiother Oncol. 2017;124(3):403-410. doi: 10.1016/j.radonc.2017.05.009.
- Meng J, Li P, Zhang Q, Yang Z, Fu S. A radiosensitivity gene signature in predicting glioma prognostic via EMT pathway. Oncotarget. 2014;5(13):4683-4693. doi: 10.18632/oncotarget.2088.
- Liu N, Boohaker RJ, Jiang C, Boohaker JR, Xu B. A radiosensitivity MiRNA signature validated by the TCGA database for head and neck squamous cell carcinomas. Oncotarget. 2015;6(33):34649-34657. doi: 10.18632/oncotarget.5299.
- Foy JP, Bazire L, Ortiz-Cuaran S, et al. A 13-gene expression-based radioresistance score highlights the heterogeneity in the response to radiation therapy across HPV-negative HNSCC molecular subtypes. BMC Med. 2017;15(1):165. doi: 10.1186/s12916-017-0929-y.
- Schmidt S, Linge A, Zwanenburg A, et al. Development and validation of a gene signature for patients with head and neck carcinomas treated by postoperative radio(chemo)therapy. Clin Cancer Res. 2018;24(6):1364-1374. doi: 10.1158/1078-0432.CCR-17-2345.
- Tang Z, Zeng Q, Li Y, et al. Development of a radiosensitivity gene signature for patients with soft tissue sarcoma. Oncotarget. 2017;8(16):27428-27439. doi: 10.18632/oncotarget.16194.
- Rajkumar T, Vijayalakshmi N, Sabitha K, et al. A 7 gene expression score predicts for radiation response in cancer cervix. BMC Cancer. 2009;9:365. doi: 10.1186/1471-2407-9-365.
- Sjostrom M, Staaf J, Eden P, et al. Identification and validation of single-sample breast cancer radiosensitivity gene expression predictors. Breast Cancer Res. 2018;20(1):64. doi: 10.1186/s13058-018-0978-y.
- Zhou J, Wu X, Li G, et al. Prediction of radiosensitive patients with gastric cancer by developing gene signature. Int J Oncol. 2017;51(4):1067-1076. doi: 10.3892/ijo.2017.4107.
- Eschrich SA, Fulp WJ, Pawitan Y, et al. Validation of a radiosensitivity molecular signature in breast cancer. Clin Cancer Res. 2012;18(18):5134-5143. doi: 10.1158/1078-0432.CCR-12-0891.
- Strom T, Hoffe SE, Fulp W, et al. Radiosensitivity index predicts for survival with adjuvant radiation in resectable pancreatic cancer. Radiother Oncol. 2015;117(1):159-164. doi: 10.1016/j.radonc.2015.07.018.
- Ahmed KA, Chinnaiyan P, Fulp WJ, Eschrich S, Torres-Roca JF, Caudell JJ. The radiosensitivity index predicts for overall survival in glioblastoma. Oncotarget. 2015;6(33):34414-34422. doi: 10.18632/oncotarget.5437.
- Strom T, Torres-Roca JF, Parekh A, et al. Regional radiation therapy impacts outcome for node-positive cutaneous melanoma. J Natl Compr Canc Netw. 2017;15(4):473-482. doi: 10.6004/jnccn.2017.0047.
- Torres-Roca JF, Fulp WJ, Caudell JJ, et al. Integration of a radiosensitivity molecular signature into the assessment of local recurrence risk in breast cancer. Int J Radiat Oncol Biol Phys. 2015;93(3):631-638. doi: 10.1016/j.ijrobp.2015.06.021.
- Carvalho S, Leijenaar RTH, Troost EGC, et al. 18F-fluorodeoxyglucose positron-emission tomography (FDG-PET)-Radiomics of metastatic lymph nodes and primary tumor in non-small cell lung cancer (NSCLC) - A prospective externally validated study. PLoS One. 2018;13(3):e0192859. doi: 10.1371/journal.pone.0192859.
- Chen T, Li M, Gu Y, et al. Prostate cancer differentiation and aggressiveness: assessment with a radiomic-based model vs. PI-RADS v2. J Magn Reson Imaging. 2019;49(3):875-884. doi: 10.1002/jmri.26243.
- Fave X, Zhang L, Yang J, et al. Delta-radiomics features for the prediction of patient outcomes in non-small cell lung cancer. Sci Rep. 2017;7(1):588. doi: 10.1038/s41598-017-00665-z.
- Gnep K, Fargeas A, Gutierrez-Carvajal RE, et al. Haralick textural features on T2 -weighted MRI are associated with biochemical recurrence following radiotherapy for peripheral zone prostate cancer. J Magn Reson Imaging. 2017;45(1):103-117. doi: 10.1002/jmri.25335.
- Grossmann P, Narayan V, Chang K, et al. Quantitative imaging biomarkers for risk stratification of patients with recurrent glioblastoma treated with bevacizumab. Neuro Oncol. 2017;19(12):1688-1697. doi: 10.1093/neuonc/nox092.
- Jeon SH, Song C, Chie EK, et al. Delta-radiomics signature predicts treatment outcomes after preoperative chemoradiotherapy and surgery in rectal cancer. Radiat Oncol. 2019;14(1):43. doi: 10.1186/s13014-019-1246-8.
- Lao J, Chen Y, Li ZC, et al. A Deep Learning-Based Radiomics Model for Prediction of Survival in Glioblastoma Multiforme. Sci Rep. 2017;7(1):10353. doi: 10.1038/s41598-017-10649-8.
- Li Q, Kim J, Balagurunathan Y, et al. CT imaging features associated with recurrence in non-small cell lung cancer patients after stereotactic body radiotherapy. RadiatOncol. 2017;12(1):158. doi: 10.1186/s13014-017-0892-y.
- Oikonomou A, Khalvati F, Tyrrell PN, et al. Radiomics analysis at PET/CT contributes to prognosis of recurrence and survival in lung cancer treated with stereotactic body radiotherapy. Sci Rep. 2018;8(1):4003. doi: 10.1038/s41598-018-22357-y.
- Vallieres M, Kay-Rivest E, Perrin LJ, et al. Radiomics strategies for risk assessment of tumour failure in head-and-neck cancer. Sci Rep. 2017;7(1):10117. doi: 10.1038/s41598-017-10371-5.
- Yip C, Landau D, Kozarski R, et al. Primary esophageal cancer: heterogeneity as potential prognostic biomarker in patients treated with definitive chemotherapy and radiation therapy. Radiology. 2014;270(1):141-148. doi: 10.1148/radiol.13122869.
- Zhou M, Hall L, Goldgof D, et al. Radiologically defined ecological dynamics and clinical outcomes in glioblastoma multiforme: preliminary results. Transl Oncol. 2014;7(1):5-13. doi: 10.1593/tlo.13730.
- Lucia F, Visvikis D, Desseroit MC, et al. Prediction of outcome using pretreatment (18)F-FDG PET/CT and MRI radiomics in locally advanced cervical cancer treated with chemoradiotherapy. Eur J Nucl Med Mol Imaging. 2018;45(5):768-786. doi: 10.1007/s00259-017-3898-7.
- Bak SH, Park H, Lee HY, et al. Imaging genotyping of functional signaling pathways in lung squamous cell carcinoma using a radiomics approach. Sci Rep. 2018;8(1):3284. doi: 10.1038/s41598-018-21706-1.
- Osman SOS, Leijenaar RTH, Cole AJ, et al. Computed tomography-based radiomics for risk stratification in prostate cancer. Int J Radiat Oncol Biol Phys. 2019;105(2):448-456. doi: 10.1016/j.ijrobp.2019.06.2504.
- Zhao L, Gong J, Xi Y, et al. MRI-based radiomics nomogram may predict the response to induction chemotherapy and survival in locally advanced nasopharyngeal carcinoma. Eur Radiol. 2019. doi: 10.1007/s00330-019-06211-x.
- ozzi L, Dinapoli N, Fogliata A, et al. Radiomics based analysis to predict local control and survival in hepatocellular carcinoma patients treated with volumetric modulated arc therapy. BMC Cancer. 2017;17(1):829. doi: 10.1186/s12885-017-3847-7.
- Kocak B, Durmaz ES, Ates E, Ulusan MB. Radiogenomics in clear cell renal cell carcinoma: machine learning-based high-dimensional quantitative CT texture analysis in predicting PBRM1 mutation status. AJR Am J Roentgenol. 2019; 212(3):W55-W63. doi: 10.2214/AJR.18.20443.
- Stoyanova R, Pollack A, Takhar M, et al. Association of multiparametric MRI quantitative imaging features with prostate cancer gene expression in MRI-targeted prostate biopsies. Oncotarget. 2016;7(33):53362-53376. doi: 10.18632/oncotarget.10523.
- Ma W, Ji Y, Qi L, Guo X, Jian X, Liu P. Breast cancer Ki67 expression prediction by DCE-MRI radiomics features. Clin Radiol. 2018;73(10):909 e901-909 e905. doi: 10.1016/j.crad.2018.05.027.
- Wang J, Kato F, Oyama-Manabe N, et al. Identifying triple-negative breast cancer using background parenchymal enhancement heterogeneity on dynamic contrast-enhanced MRI: a pilot radiomics study. PLoS One. 2015;10(11):e0143308. doi: 10.1371/journal.pone.0143308.
- Yeh AC, Li H, Zhu Y, et al. Radiogenomics of breast cancer using dynamic contrast enhanced MRI and gene expression profiling. Cancer Imaging. 2019;19(1):48. doi: 10.1186/s40644-019-0233-5.
- Abdollahi H, Mahdavi SR, Shiri I, Mofid B, Bakhshandeh M, Rahmani K. Magnetic resonance imaging radiomic feature analysis of radiation-induced femoral head changes in prostate cancer radiotherapy. J Cancer Res Ther. 2019;15(Supplement):S11-S19. doi: 10.4103/jcrt.JCRT_172_18.
- Colen RR, Fujii T, Bilen MA, et al. Radiomics to predict immunotherapy-induced pneumonitis: proof of concept. Invest New Drugs. 2018;36(4):601-607. doi: 10.1007/s10637-017-0524-2.
- Dong Y, Feng Q, Yang W, et al. Preoperative prediction of sentinel lymph node metastasis in breast cancer based on radiomics of T2-weighted fat-suppression and diffusion-weighted MRI. Eur Radiol. Feb 2018;28(2):582-591. doi: 10.1007/s00330-017-5005-7.
- McGarry SD, Bukowy JD, Iczkowski KA, et al. Gleason probability maps: a radiomics tool for mapping prostate cancer likelihood in MRI space. Tomography. 2019;5(1):127-134. doi: 10.18383/j.tom.2018.00033.
- Shen C, Liu Z, Wang Z, et al. Building CT radiomics based nomogram for preoperative esophageal cancer patients lymph node metastasis prediction. Transl Oncol. 2018;11(3):815-824. doi: 10.1016/j.tranon.2018.04.005.
- Wu S, Zheng J, Li Y, et al. A Radiomics nomogram for the preoperative prediction of lymph node metastasis in bladder cancer. Clin Cancer Res. 2017;23(22):6904-6911. doi: 10.1158/1078-0432.CCR-17-1510.
- Yu H, Caldwell C, Mah K, Mozeg D. Coregistered FDG PET/CT-based textural characterization of head and neck cancer for radiation treatment planning. IEEE Trans Med Imaging. 2009;28(3):374-383. doi: 10.1109/TMI.2008.2004425.
- Lohmann P, Stoffels G, Ceccon G, et al. Radiation injury vs. recurrent brain metastasis: combining textural feature radiomics analysis and standard parameters may increase (18)F-FET PET accuracy without dynamic scans. Eur Radiol. 2017;27(7):2916-2927. doi: 10.1007/s00330-016-4638-2.
- Bowen SR, Yuh WTC, Hippe DS, et al. Tumor radiomic heterogeneity: Multiparametric functional imaging to characterize variability and predict response following cervical cancer radiation therapy. J Magn Reson Imaging. 2018;47(5):1388-1396. doi: 10.1002/jmri.25874.
- Cui Y, Yang X, Shi Z, et al. Radiomics analysis of multiparametric MRI for prediction of pathological complete response to neoadjuvant chemoradiotherapy in locally advanced rectal cancer. Eur Radiol. 2019;29(3):1211-1220. doi: 10.1007/s00330-018-5683-9.
- Hou Z, Yang Y, Li S, et al. Radiomic analysis using contrast-enhanced CT: predict treatment response to pulsed low dose rate radiotherapy in gastric carcinoma with abdominal cavity metastasis. Quant Imaging Med Surg. 2018;8(4):410-420. doi: 10.21037/qims.2018.05.01.
- Paul J, Yang C, Wu H, et al. Early Assessment of treatment responses during radiation therapy for lung cancer using quantitative analysis of daily computed tomography. Int J Radiat Oncol Biol Phys. 2017;98(2):463-472. doi: 10.1016/j.ijrobp.2017.02.032.
- Wu J, Gong G, Cui Y, Li R. Intratumor partitioning and texture analysis of dynamic contrast-enhanced (DCE)-MRI identifies relevant tumor subregions to predict pathological response of breast cancer to neoadjuvant chemotherapy. J Magn Reson Imaging. 2016;44(5):1107-1115. doi: 10.1002/jmri.25279.
- Eschrich SA, Pramana J, Zhang H, et al. A gene expression model of intrinsic tumor radiosensitivity: prediction of response and prognosis after chemoradiation. Int J Radiat Oncol Biol Phys. 2009;75(2):489-496. doi: 10.1016/j.ijrobp.2009.06.014.
- Vallieres M, Zwanenburg A, Badic B, Cheze Le Rest C, Visvikis D, Hatt M. Responsible radiomics research for faster clinical translation. J Nucl Med. 2018;59(2):189-193. doi: 10.2967/jnumed.117.200501.
- Sanduleanu S, Woodruff HC, de Jong EEC, et al. Tracking tumor biology with radiomics: a systematic review utilizing a radiomics quality score. Radiother Oncol. 2018;127(3):349-360. doi: 10.1016/j.radonc.2018.03.033.
- Berger MF, Mardis ER. The emerging clinical relevance of genomics in cancer medicine. Nat Rev Clin Oncol. 2018;15(6):353-365. doi: 10.1038/s41571-018-0002-6.
- Speers C, Pierce LJ. Molecular signatures of radiation response in breast cancer: towards personalized decision-making in radiation treatment. Int J Breast Cancer. 2017;4279724. doi: 10.1155/2017/4279724.
- Cushman TR, Caetano MS, Welsh JW, Verma V. Overview of ongoing clinical trials investigating combined radiotherapy and immunotherapy. Immunotherapy. 2018;10(10):851-850. doi: 10.2217/imt-2018-0019.
Citation
Grass GD, Mills MN, Scott JG, Eschrich SA, Torres-Roca J. Genomics and radiomics: Tools to see the unseen to personalize radiation therapy. Appl Radiat Oncol. 2019;(4):9-22.
December 28, 2019