Integrating AI and Human Expertise: Exploring the Role of Radiomics in Multidisciplinary Tumor Boards
Affiliations
- Medical College, Karachi Medical and Dental College, Karachi, Pakistan
- Department of Oncology, Section Radiation Oncology, Aga Khan University Hospital, Karachi, Pakistan
- Department of Oncology, McGill University, Montreal, Canada
- Department of Medical Oncology, Shaukat Khanum Memorial Cancer Hospital and Research Centre, Karachi, Pakistan
Abstract
In the ever-evolving landscape of oncology, the integration of artificial intelligence (AI) with radiomics has transformed the decision-making processes within multidisciplinary tumor boards (MDTs). MDTs serve as forums where specialists collaboratively discuss and recommend comprehensive treatment plans for patients with cancer, considering various clinical perspectives. This narrative review explores the synergistic relationship between AI and radiomics in oncology decision-making. We discuss the fundamentals of AI-powered radiomics, its workflow, and the role of radiomic features. Moreover, we delve into the integration of AI in radiology, radiation oncology, and medical oncology, emphasizing its impact on MDT decision-making, treatment planning, and predicting treatment responses, prognosis, and disease progression. Furthermore, we highlight the role of machine learning algorithms and their impact on MDT decision-making. We discuss the challenges and future implications of AI-driven radiomics in MDTs, considering ethical, financial, and regulatory aspects. Finally, we emphasize the transformative potential of AI-powered radiomics in reshaping oncology decision-making, facilitating more personalized and effective treatment strategies within MDTs.
Introduction
In the ever-evolving field of oncology, the utilization of artificial intelligence (AI) with radiomics has paved an integral path of innovation, redefining decision-making processes toward novel precision within multidisciplinary tumor boards (MDTs). MDTs are forums where specialists collaborate to collectively discuss and recommend a comprehensive treatment plan for patients with cancer.1 The collaborative nature of MDTs plays a key role in addressing the complexities of cancer care, considering various perspectives of clinical presentation, ranging from surgical oncology to medical and radiation oncology backed with radiological and pathological support.
Studies such as that by Kočo et al emphasize the significance of MDTs in improving diagnostic accuracy, treatment planning, and patient outcomes. Active discussions within MDTs allow for a holistic understanding of individual cases, considering not only the clinical aspects but also the unique clinical presentation of each patient, thereby offering an individualized approach for each patient.2 As stated by Meng et al, incorporation of radiomics can be a key component of precision therapy and an innovative approach to the collective decision-making process in MDTs, resulting in more personalized and tailored treatment plans, avoidance of treatment delays due to invasive procedures, and reflecting a unique integration of diverse knowledge in enhancing patient-centric disease management.3
The background of the transformative shift of cancer care toward AI lies in the intrinsic challenges posed by conventional oncological approaches, which are marked by limitations in data processing speed, pattern recognition, and personalized insights. The combination of AI and radiomics, a field focused on extracting quantitative information from medical images, holds immense promise in addressing these challenges. Embracing AI-powered radiomics in oncology decision-making is evident in its ability to swiftly process vast and complex imaging datasets, facilitating an understanding of subtle patterns and variations within tumors.4
This narrative review aims to provide a comprehensive overview of the synergistic relationship between AI and radiomics in oncology. By delving into the background issues of traditional methodologies and interpreting the transformative potential offered by AI-driven radiomics, this communication seeks to bridge the gap between the theoretical insights and practical implications. Through a critical review of the current landscape, we aim to emphasize the significance of this combination in reshaping the decision-making processes in MDTs, discussing its challenges and, emphasizing the need for a paradigm shift toward AI-powered radiomic applications in multidisciplinary clinical practice.
Fundamentals of AI-Powered Radiomics
Understanding Radiomic Workflow in Oncology
Radiomics can be defined as the extraction of data from medical imaging and its practical application within the field of oncology with the intent to improve diagnosis, prognostication, and devising treatment recommendation within the MDT setting, with the goal of delivering timely precise treatment. The role of radiomics in oncology revolves around a spectrum of properties, ranging from shape, size, texture, and intensity to a comprehensive depiction of tumor heterogeneity. The rationale behind incorporating radiomics lies in the notion that subtle patterns within medical images may contain high-dimensional data about tumor biology, behavior, response to treatment, and prognosis, which, once utilized, can translate into transformative oncological patient care.4
Radiomic workflow is a collaborative effort involving radiologists, data scientists, and imaging experts. Its process involves tumor segmentation, preprocessing of acquired images, representative feature extraction, model development, and validation ( Figure 1 ). Extracted features include signal intensity and spatial relationships among pixels within gross tumor volume (GTV), followed by validation of proposed data through a cross-check carried out on the dataset.4
Radiomic workflow diagram.
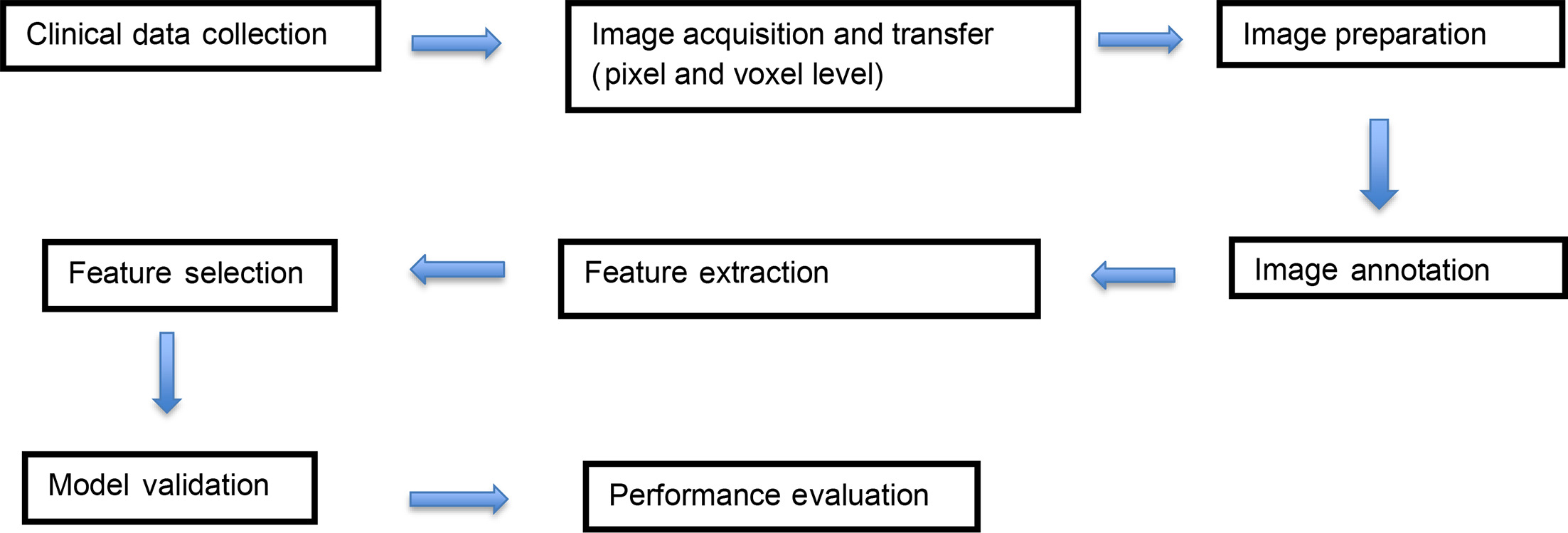
In a landmark study by Lambin et al, radiomics was referred to as a bridge between medical imaging and personalized management, highlighting its potential to decipher the complexities of tumor biology.5
Radiomic Features
A study by Kocak et al provided a detailed algorithm highlighting the proficiency of AI algorithms in smoothly processing extensive imaging datasets, facilitating a rapid and comprehensive evaluation of radiomic features. Features such as data curation and preprocessing strategies such as pixel resampling using the Medical Image Processing, Analysis, and Visualization (MIPAV) software, and 3DSlicer, play an integral role in optimizing the extraction of quantitative information.6
Furthermore, machine learning and deep learning—two key concepts in radiomics—are the AI subfields revolutionizing medical diagnostics, treatment, and research. A machine learning process encompasses various techniques, such as decision trees (DTs), random forests (RFs), and support vector machines (SVMs), for classifying tumor types in medical imaging and neural networks, aiding in diagnosis and drug discovery by identifying various therapeutic targets.
Each model’s practical application is grounded in an understanding of its strengths and practicality, with a few relevant practical scenarios listed as follows:
Decision trees : These are ideal when interpretability is crucial, suitable for small- to medium-sized datasets, and useful for understanding feature importance. For instance, while combining DTs can yield valuable insights, the complexity increases and consensus varies depending on the specific criteria and number of DTs compared in the case of prostatic adenocarcinoma.7
Random forests : These are effective for high-dimensional data, providing robustness and better generalization, and capable of handling larger datasets. For instance, risk prediction models via RFs in breast cancer prognosis and treatment have shown superior results in prediction accuracy compared with traditional regression models.8
Support vector machines : These models are best for small- to medium-sized datasets, particularly for binary classification problems, and when there is a clear margin of separation between classes. For instance, SVM models have shown 90% accuracy, 80% sensitivity, and 80% specificity in the diagnosis of early prostate cancer.9
Neural networks : These networks are suitable for large datasets and complex patterns, excelling in tasks involving image, audio, or text data, where deep learning can utilize vast amounts of data for feature learning and pattern recognition.
Deep learning, a subset of machine learning, focuses on deep neural networks. Examples are convolutional neural networks for detecting anomalies in x-rays, MRIs, and CT scans; recurrent neural networks for analyzing wearable device data to monitor patients’ vitals; and deep reinforcement learning for optimizing personalized treatment plans. Traditional machine learning relies on manual feature engineering using simpler models that are suitable for smaller datasets and easier to interpret. It is particularly effective for structured data. In contrast, deep learning performs automatic feature extraction with more complex models that require large datasets and significant computational power. These models are harder to interpret and are often considered “black boxes,” but are highly effective for unstructured and high-dimensional data.10
AI Integration in Radiology, Radiation Oncology, and Medical Oncology
AI can be defined as the simulation of human thinking, logical reasoning, problem solving, and deep learning processes by machines, particularly computers. AI in radiomics can be defined as advanced algorithms formulating predictions based on radiological data incorporated into the software. This integration of AI with radiomics may further amplify the recommended yield of MDT with the help of vast and complex datasets in oncology, the key revolution being in the field of neuro-oncology, where AI-incorporated radiomics and radiogenomic tools can potentially stratify various tumors based on radiological data only. This accelerated analysis can empower MDTs with information, enabling a thorough understanding of tumor characteristics and biology, which may not be readily apparent through conventional means, including biopsy for challenging locations. Examples include intracranial diffuse lesions (primary central nervous system lymphoma), or anatomically challenging regions (including malignancies involving the brain stem, or to differentiate between postradiation necrosis vs recurrence.11
AI applications regarding radiomics are not only limited to radiological image interpretation but also to their use in radiation oncology. They have facilitated the optimization of workflow, including auto segmentation, auto contouring, dose optimization, radiation dose tracking, and automated quality assurance checks. However, prior to clinical implementation, a mandatory multidisciplinary evaluation of credibility preceded by the data entered is mandated. Therefore, AI, when combined with clinical decision support systems (CDSS), can greatly improve clinical and radiological workflows.12
Furthermore, systems such as RadCloud have standardized radiomic feature extraction and made it easier to integrate deep-learning-based image data from medical records in coherence with AI algorithms to incorporate into clinical research as well. A retrospective analysis of 139 patients done to predict breast tumors on mammograms and MRI images (built via RadCloud and designed to assess HER2/neu expression) predicted treatment response to targeted agents, including trastuzumab and pertuzumab, and estimated Ki-67 index. Another model utilized dynamic contrast-enhanced MRI to predict the probability of axillary lymph node metastasis in invasive ductal carcinoma of the breast. Likewise, a dataset of 165 patients with locally advanced rectal carcinoma was modeled to predict treatment response after neoadjuvant chemoradiation to guide subsequent treatment.13
In a multicenter study by Liu et al, MRI sequence data were integrated to predict pathological complete response to neoadjuvant therapy in patients with breast cancer. The study included radiological features of 586 patients, with a score involving features of 13,950 datasets. Quantitative analyses demonstrate it as a promising predictive tool for assessing tumor response in patients with locally advanced breast cancer, highlighting its potential and practical utility within MDTs.14
As AI continues to shape the medical imaging landscape, its integration into the field of urological oncology has led to impressive results. For prostate cancer diagnostics, machine learning has shown promise in refining clinically significant lesion detection, with some success in deciphering ambiguous lesions on multiparametric MRI (mpMRI). For kidney cancer, radiomics has emerged as a valuable tool for better distinguishing between benign and malignant renal masses and predicting tumor behavior from CT or MRI scans. Meanwhile, regarding bladder cancer, there is a burgeoning emphasis on the prediction of muscle invasive cancer and forecasting disease trajectory.15
Arimura et al discussed the concept of radiomics and its application in precision medicine in radiation therapy (RT), emphasizing the use of AI and machine learning algorithms to predict outcomes and toxicity for individual patients based on radiomic biomarkers extracted from medical images. It highlights the importance of radiomics in stratifying patients into subtypes based on imaging biomarkers to improve decision-making in precision medicine and treatment strategies, as well as the potential to avoid complications caused by invasive biopsies.16
The application of radiomics and machine learning in head and neck cancers has been further elaborated by Peng et al, emphasizing the extraction of quantitative imaging features to assess tumors and personalize diagnosis and treatment plans, with a focus on predicting prognosis indicators like progression-free survival, overall survival (OS), and distant metastasis. It also mentions the potential of radiomics in laying the foundation for personalized treatment and sequential treatment of tumors in otolaryngology patients.17
Abbasi et al highlight the promising association between radiological data and genetic factors and how the concept of virtual biopsies facilitated by radiomics may challenge traditional management, offering noninvasive tumor evaluation. The integration of radiomics and AI holds promise for personalized oncology, though data maturity remains a subject of ongoing exploration.18
The Role of Machine Learning Algorithms and Its Impact on MDT Decision-Making: Case Studies
Machine learning and deep learning algorithms play distinct, yet synergistic roles in the context of AI-driven radiomics. Machine learning processes involve the classification and categorization of radiomic features that help in ascertaining complex patterns of diagnostically challenging tumors. Cobo et al proposed guidelines for generalization and widespread application of radiomic features in the MDT setting, including metadata information availability, anonymization of patient demographics, and generalized availability of data to be reproduced and applied, along with the identification of potential bias, if any, during segmentation of radiomic features.19
How to handle metadata and its clinical application was described by Vial et al, who elaborated the role of deep learning via radiomics and its impact on cancer-specific predictive modeling, termed the probabilistic max-pooling technique, which has been shown to achieve excellent accuracy for numerous pattern recognition tasks for big image sets by reducing the amount of data to be analyzed and increasing efficiency for outcome prediction.20 Chaddad et al addressed the fusion of deep learning and mpMRI to personalize the diagnosis and suggest risk stratification of prostate cancer compared with conventional transurethral resection of the prostate.21 The interplay of these algorithms into the radiomic workflow enhances the precision of tumor analyses, paving the way for more informed decision-making within an MTD setting.
The time required for a single patient to undergo full radiomic processing — including MRI DICOM prenormalization, tumor segmentation on multiple MRI sequences, radiomic feature extraction, and integration of radiomic and other clinical/molecular outputs into the selected model — can vary significantly, typically ranging from 3 to 8 hours. MRI DICOM prenormalization usually takes 15 minutes to 1 hour, depending on the need for manual adjustments. Tumor segmentation, which can be manual, semi-automatic, or automatic, generally requires 1 to 3 hours. Radiomic feature extraction often takes 30 minutes to 2 hours, influenced by the number and complexity of features extracted. Finally, integrating radiomic data with clinical and molecular outputs into the selected model usually needs 1 to 2 hours. The level of automation, quality of input data, operator experience, and computational resources significantly impact these time estimates.22
Table 1 highlights a few key studies incorporating radiomics and AI to enhance the workflow of MDT.
Key Studies Proposing Models for Incorporating AI and Radiomics in MDT Workflow
STUDY FOCUS | RESEARCH HYPOTHESIS | AI-DRIVEN RADIOMICS |
---|---|---|
Glioma radiomics23 | Explores AI-based MRI radiomics for glioma classification and prognosis, emphasizing feature extraction and model construction | CNN, RNN, LSTM, VASARI radiomic features |
Lung cancer radiomics24 | Evaluates AI applications in lung cancer screening, diagnosis, and treatment, integrating imaging and clinical data | Multiview ConvNets, DLAD |
Radiomics in predicting nodule (benign vs malignant)25 | Investigates radiomics-based classification of malignant vs benign tumors | ICCs, Wilcoxon rank-sum test, ComBat harmonization, LASSO |
Abbreviations : AI = artificial intelligence, CNN = convolutional neural network, RNN = recurrent neural network, LSTM = long short-term memory, VASARI = Visually AcceSAable Rembrandt Images, ConvNets = convolutional networks, DLAD = deep-learning-based automatic detection algorithm, ICCs = intraclass correlation coefficients, LASSO = least absolute shrinkage and selection operator.
Radiation Therapy Treatment Planning and AI
One of the key breakthroughs lies in the optimization of treatment planning through the incorporation of radiomic features. Regarding radiation oncology, Shiradkar et al developed a computational framework known as radiomic-based TRaP for prostate cancer treatment planning based on MRI radiomic features. This framework comprises 3 distinct steps: radiomic feature-based detection of prostatic cancer on mpMRI data added via deep learning feature and segmentation; deformable co-registration of planning scan with diagnostic mpMRI for target and organ at risk of auto contouring; and radiomic-based planning for external-beam radiation therapy or brachytherapy. Their work showed that while maintaining normal target dose optimization objectives, radiomics-based planning minimizes the radiation dosage to the bladder and rectum.26
Treatment Response, Prognosis, and Disease Progression
Radiomics has been proven to be instrumental in predicting treatment responses. The identification of specific radiomic imaging biomarkers allows for the early assessment of a patient’s likelihood of responding to therapy, enabling clinicians to customize treatment strategies.
Chan et al stratified early breast cancer patients into low-risk and high-risk for treatment failure in the analysis of 563 patients, utilizing the concept of Eigen tumors (a tool for feature selection). This concept involves image feature acquisition from pretreatment tumors and a predictive model of post-treatment response constructed using survival analysis showing a significant difference between estrogen receptor-positive and HER2/neu-negative tumors.27 Such methods of quantification optimize MDTs by offering personalized insights for greater efficacy and targeted cancer treatment plans.
After studying tumor properties, AI-driven radiomic algorithms demonstrated superior capabilities in recognizing subtle patterns indicative of disease progression and metastasis.
Tang et al proposed a radiomic model for prognosis prediction in non-small cell lung cancer (NSCLC). This model integrates radiomic features derived from RT planning images with key clinical information, including age, gender, histology, and tumor stage. CT images of 422 patients with NSCLC were sourced from The Cancer Imaging Archive, with radiomic features extracted from the GTVs. Five learning algorithms were incorporated: DTs, RFs, extreme boost, SVMs, and generalized linear model. This combined radiomic model compared with the radiomic model predicted 1-year survival with greater accuracy of area under the curve (AUC) of 0.941, 0.856, and 0.949, respectively.28
Enhancing Collaborative Decision-Making in MDTs
Integrating Radiomics Into MDT Workflows
The introduction of radiomics into MDT workflows ( Figure 2 ) has become a promising tool in the decision-making landscape. Radiomics adds a layer of precision to the collective understanding of tumor characteristics, providing a comprehensive analysis of medical images, extracting phenotypic features that reflect cancer prognosis. Along with radiomics, radiogenomics and radioimmunomics are other clinical data that can be incorporated to increase the predictive capacity and accuracy of MDT, but they warrant further research and generalization.29
The basic workflow of radiomic incorporation in multidisciplinary tumor boards (MDTs).
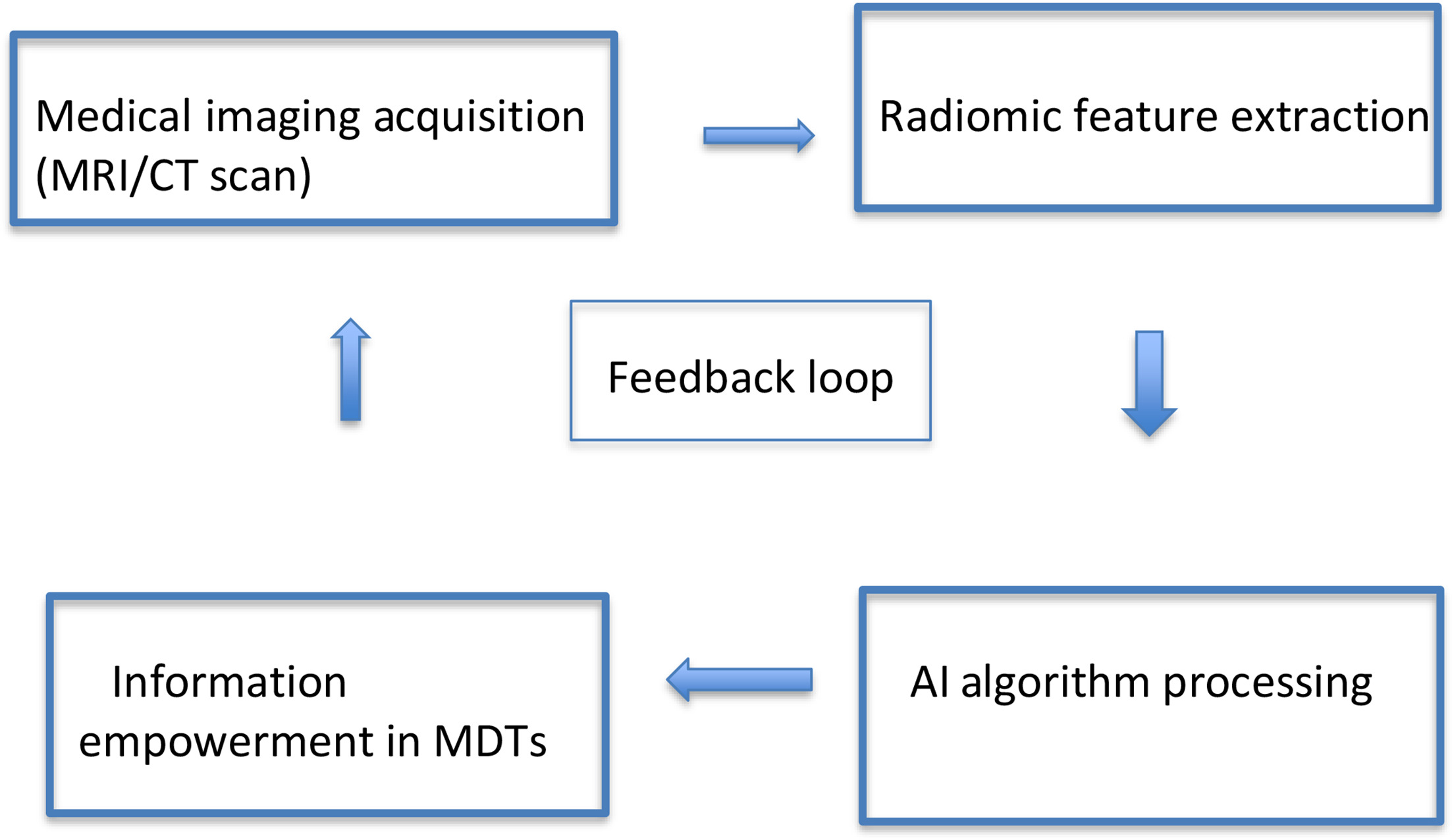
By seamlessly incorporating radiomic analyses into the MDT workflow, clinicians gain access to a source of quantitative data, augmenting their ability to tailor treatment strategies based on the unique radiomic profile of each patient’s tumor in a noninvasive and individualized manner.30
AI Technology and Its Implication in the Real World: Applications
Radiomic features can be influenced by specific variations in images, impacting their ability to be widely applicable and reproducible. Moreover, the clinical utility of radiomics faces obstacles such as small study cohorts, insufficient external dataset validation, and inconsistent feature calculation methods. Despite these challenges, the integration of AI and radiomics holds immense promise in both clinical trials and real-world scenarios, with ongoing endeavors to mitigate these shortcomings. Key concerns include the lack of standardization and generalizability in radiomics findings, inadequate data quality control, issues with repeatability and reproducibility, imbalances in databases, and the risk of model overfitting. To ensure robust and transferable results across different patient cohorts, radiomic procedures must meticulously address these pitfalls and challenges.
Fusco et al discussed the critical issues in radiomics research, emphasizing the need for high-quality images with consistent protocols, adequate and complete datasets, and distinct training and validation sets. They highlighted the problem of class imbalances and the necessity of reporting class-wise accuracy, sensitivity, and specificity.
Overfitting is a major concern when models include too many parameters, leading to the inclusion of irrelevant features and poor generalization. To prevent this, Fusco et al suggested using smoothing techniques and reducing input parameters, alongside validating models with separate datasets. They also mention the issue of underfitting, where overly simplistic models fail to classify data accurately, stressing the need for balanced model complexity.31
Another analysis involving advancements in AI-based radiomics for lung cancer immunotherapy deduced that current studies, while promising, were too preliminary and lacked methodological rigor, hindering clinical adoption. Most studies were observational rather than interventional and suggest further research with large cohorts, rigorous testing, validation, and methodological improvements to make these tools clinically viable for selecting patients with lung cancer for immunotherapy.32
AI and Analysis Before and After Its Advent in Oncology
AI and radiomics have demonstrated significant benefits in oncology, outperforming traditional methods and offering new opportunities for clinical decision support. For example, an AI model surpassed radiologists and conventional models in predicting lymph node metastasis in pancreatic ductal adenocarcinoma. Regarding radio-oncology, big data processing helps improve treatment outcomes and stimulates research on radiation responses, necessitating more AI training for medical physicists.33 AI-based radiomics has enhanced clinical decision-making for patients with HCC (hepatocellular carcinoma)by improving prediction, diagnosis, and prognosis.34 Machine learning tools for nodule management show promise in reducing radiology workload through automated detection, measurement, and risk stratification of nodules. Additionally, AI-based radiomics reduces interobserver variability in medical image interpretation, offering quantitative insights often overlooked by clinicians.
Peeken et al proposed incorporating AI into CDSS to facilitate precise, personalized treatment strategies and enhanced prognostication by analyzing extensive datasets to identify effective treatment patterns. This approach enables oncologists to make more informed decisions and provides patients with a deeper understanding of their disease and treatment options, empowering them throughout their care journey. Additionally, they emphasized that the role of AI is to support rather than dictate treatment decisions, underscoring the importance of comprehensive training for health care professionals to maintain patient-centered care. The future of cancer care is likely to involve a collaborative model that combines AI’s capabilities with oncologists’ expertise, promoting informed patient decisions and personalized treatment.35 Moreover, AI’s application in liquid biopsies can offer noninvasive insights into the genetic makeup of cancers, facilitating treatment monitoring and recurrence detection.36
Such advancements suggest that AI and radiomics can revolutionize oncology care, making them more personalized, accurate, and efficient.37
Future Implications
In the realm of MDTs, the incorporation of AI-driven radiomics opens up novel opportunities for the future of precision oncology decision-making (see Figure 3 for a proposed workflow of incorporating AI in MDTs). This drives a growing trend toward personalized treatment tactics, as evidenced by various studies demonstrating the ability to decode detailed radiomic processes and modify methods of treatment. Additionally, this advancement emphasizes the individualized nature of treatments, which adapt to the particular traits of each patient’s tumor. Instantaneous combining of radiomic data enables MDTs to dynamically adjust to changing patient conditions, ensuring that treatment plans are continuously optimized. Meanwhile, a blend of multi-omics data, such as genomes, epigenetics, and radiomics, has been proven to provide a more in-depth evaluation of a patient’s risk stratification and targeted treatment options in the era of immunotherapy.
Proposed workflow of artificial intelligence (AI) incorporation in multidisciplinary tumor boards (MDTs).
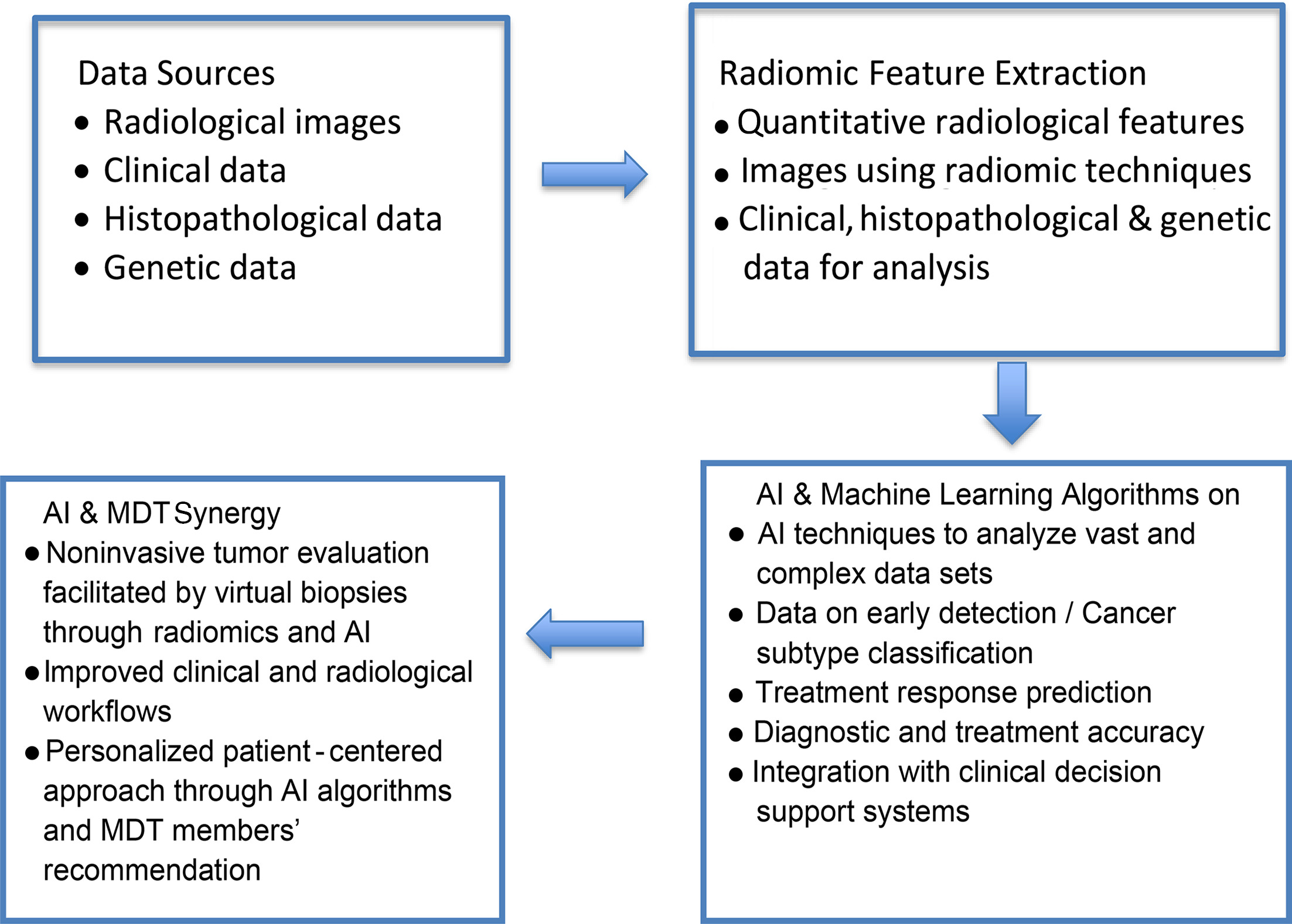
Future implications of such a scenario can be seen in patients with IDH (isocitrate dehydrogenase)-wildtype glioblastoma, where the preoperative MRI features of 516 patients were extracted in coherence with molecular classifiers (MGMT methylation status). Patients were grouped into high risk and low risk on the basis of features backed up by SVMs. The basic difference between the two cohorts was the duration of OS. This trained radiomic model (SVM) was independently evaluated and a patient-wise OS prediction was deduced. Multivariate models were generated first on the basis of clinical parameters, then by adding radiomic and molecular information. The evaluation of OS in the experimental cohort revealed AUCs of 0.78 (95% CI 0.70-0.85)/0.75 (95% CI 0.64-0.79) and 0.75 (95% CI 0.65-0.84)/0.63 (95% CI 0.52-0.71), respectively. Cox regression analysis showed a concordance index of 0.65 for clinical data compared with 0.75 for combined data (radiomics and molecular), thereby accurately predicting the OS. The authors mention that the key limitation of this study is the single-instituttion analysis of prospective data; hence, further collaboration and multi-institution analysis will strengthen SVM.38
Such developments hold potential for finding the gaps in current management, resulting in stronger decision-making. Addressing the interpretability challenge, these explainable AI models not only promote openness in decision-making processes but also build confidence among clinicians, which is imperative for collaborative decision-making in MDTs.
Unveiling the Other Perspective: Debate of Now
Ethical regulation of AI, particularly in the case of MDTs, is of paramount importance. For instance, the AI guiding physicians on the tumor board may lead to erroneous decisions influenced by AI. In such cases, accountability becomes a pressing question.
Given the novelty of these topics, their contribution to solving complex cases in patient management is of interest. However, there is a concern that they might also lead to misguided solutions since an oncologist or a radiologist has vast experience and can contemplate a scenario-based approach toward any recommendation for the patient’s management. However, AI revolves around technical data only, with the individualized approach being a challenge; hence, creating a balance between dynamic power/knowledge will be a challenge.39
Exploring the financial aspect of AI utilization, particularly in diagnostics, presents intriguing possibilities. For instance, leveraging radiomics instead of biopsy could potentially reduce testing costs. But, in contrast, it raises an important aspect regarding managing AI cost and reliability.40
Scenarios in which AI errors stem from false inputs result in inaccurate outputs raising important questions about responsibility and accountability. Moreover, current radiomic data are mostly retrospective image data, needing a centralized and robust technical support team.41
A proposed AI-integrated tumor board, along with its practical implementation, will involve several steps. Before the tumor board’s meeting, the AI system processes all relevant patient data, including medical images, pathology reports, genetic profiles, and clinical histories, and generates detailed reports highlighting the key findings, potential diagnoses, and recommended treatment options. During the meeting, these AI-generated reports are reviewed by the multidisciplinary team, supplementing their expertise and leading to more informed and comprehensive discussions. The final treatment plan should be developed collaboratively, with the AI’s recommendations serving as an additional resource to guide decision-making. The outcomes of the treatment plans will be monitored and fed back into the AI system to continuously improve its algorithms and recommendations.
Integrating AI into MTBs not only enhances diagnostic accuracy and streamlines decision-making but also personalizes treatment recommendations. While the processing and integration time required for radiomics and AI could initially pose a significant limitation in streamlining this concept, future advancements in methods and workflows hold promise for overcoming this challenge. Despite the current barriers, radiomics and AI have the potential to become invaluable tools in future tumor board settings. As methods become more refined and workflows optimized, the integration of radiomics and AI can enhance diagnostic accuracy, treatment planning, and patient outcomes in cancer care.
This collaborative approach, where AI aids rather than replaces human expertise, leverages the strengths of both technology and medical professionals to improve patient care outcomes. Fetah et al exemplify this integration, showcasing the practical benefits and potential of AI in a clinical setting, ultimately leading to timely interventions, more effective treatments, and improved outcomes for patients with cancer.40
Finally, ethical considerations, integration challenges, regulatory settings, economic elements, patient-centric beliefs, and long-term implications all highlight the need for continued research. By overcoming obstacles in these and related areas, the future prospects of AI-powered radiomics in MDTs can help advance precision oncology. This growth, in turn, can facilitate equitable and efficient implementation across the global health care arena, as MDTs serve as crucial forums for comprehensive collaborative decision-making, significantly impacting patient care and outcomes, and serving as a lifeline for patient management.41
Conclusion
The combination of radiomics and AI represents a revolutionary synergy that changes the oncology decision-making environment. This review delved into the intricate realms of AI-driven radiomics, elucidating their substantial impact on MDTs while also identifying the key challenges in solely relying on AI within the MDT context. When combined with AI algorithms’ cognitive amplification powers, radiomics incorporation into MDT workflows allows for a quantum leap in our knowledge of and approach to cancer management.
References
Citation
Shahid SF, Ali T, MBBS; Khan MH, Sheikh NA, Abbasi, AN. Integrating AI and Human Expertise: Exploring the Role of Radiomics in Multidisciplinary Tumor Boards. Appl Radiat Oncol. 2024;(2):5 - 14.
doi:10.37549/ARO-D-24-00014
June 1, 2024