Synthetic CT: Realizing the Benefits of MR-Based Treatment Planning
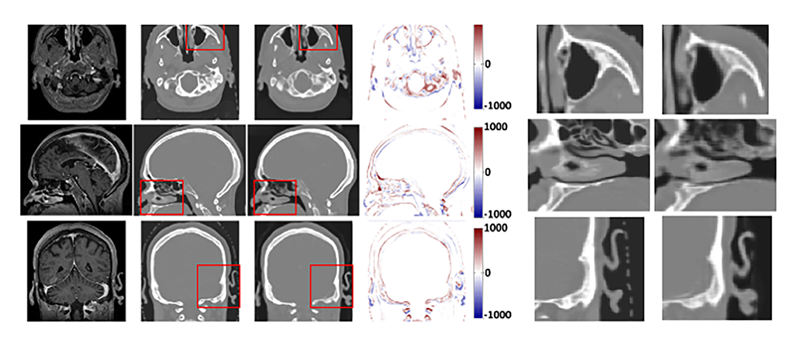
Comparison between ground truth CT and synthetic CT (sCT). From left to right, the images are MRI, CT, sCT and the difference images between CT and sCT. Three regions of interest (ROIs) are outlined by the red box. The corresponding CT and sCT on 3 directions within the ROIs are shown in the second figure (right). The display windows are [0, 500] for MRIs and [−1000 1000] for CT images. Reference: Lei Y, Harms J, Wang T, Liu Y, Shu HK, Jani AB, Curran WJ, Mao H, Liu T, Yang X. MRI-only based synthetic CT generation using dense cycle consistent generative adversarial networks. Med Phys. 2019;46(8):3565-3581. doi:10.1002/mp.13617. Epub 2019 Jun 12. Reprinted with permission.
In radiation therapy treatment planning, MRI enables effective targeting of tumors and delineation of organs at risk (OARs), while CT has been the mainstay modality for determining electron density and dose calculations. Two separate datasets introduce inherent errors into the treatment planning process, however, and often complicate or lengthen treatment planning workflow for patients who require both exams.
“When you have CT and MR separately, you must register those images together to draw a target and OARs. Because those images are obtained at separate times and sometimes different positions—with the CT taken in the treatment position—when you put them together, there are sometimes slight differences,” explains Hyeri Lee-Poprocki, PhD, radiation physicist at The Ohio State University Wexner Medical Center. “When those images are registered, there are inherent errors due to positions or anatomical changes because there might be up to a week or 2 between the image acquisitions.”
Alternatively, using MRI data, radiation therapy teams are creating synthetic CTs for patient positioning and radiation dose calculation, an approach once performed through manual calculations now achievable via various technical developments. MRI as a single modality in treatment planning enables excellent soft-tissue contrast, eliminates registration difficulties, and can generate density information, all without radiation exposure and exam-related delays for the patient. Research on synthetic CT production from MRIs has occurred mostly in the treatment of tumors of the brain, an optimal site for application due to the lack of motion, according to Xiaofeng Yang, PhD, DABR, associate professor and vice chair for medical physics research in the Department of Radiation Oncology at Emory University School of Medicine. Head and neck, abdomen and pelvis are also sites for which synthetic CT may be utilized, he notes.
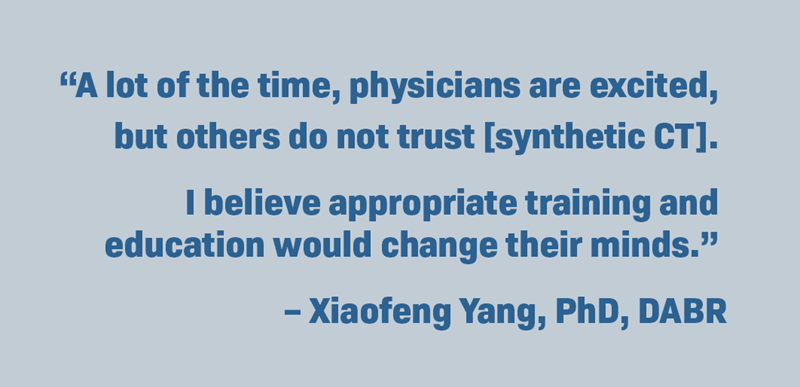
“The motivations behind MR-based treatment planning are many,” says Dr. Yang. “We can spare the patient the CT radiation exposure, simplify clinical workflow, reduce medical costs, and eliminate systematic MR-CT co-registration errors.”
As synthetic CT from MRIs begins to replace conventional CT simulation as part of traditional clinical treatment planning, workflows will evolve, requiring more research, training, and additional collaboration between radiology and radiation oncology departments and among treatment planning teams. Much of the research on synthetic CT production from MRIs using different algorithms has its own limitations, but experts expect better results with an artificial intelligence (AI) approach, says Dr. Lee-Poprocki.
“This is where the physicists come in, to take responsibility for consistency and ensure clinical implementation of synthetic CT for treatment planning is correct and safe,” he says.
Dr. Yang adds that clinicians must collaborate with physicists and dosimetrists for each patient treatment to determine if the synthetic CT is high enough quality for setup and dose calculation.
“To get the benefits of synthetic CT in radiation therapy, the whole group needs to be on the same page,” says Dr. Yang. “In the traditional workflow, physicians don’t have responsibility to check because we use a planning CT. When we apply AI technology for clinical use, the problem is we don’t have very clear, detailed guidelines about the tolerance and what’s good enough quality compared with the planning CT.”
This is one of several kinks that need to be straightened out in synthetic CT use, a byproduct of the relatively new technology application, agrees Dr. Lee-Poprocki.
Development and Research
The concept of producing synthetic CT from MR has existed for years. More than a decade ago, Dr. Yang performed segmentation-based and atlas-based methods to generate MRI-based synthetic CT for PET attenuation correction of a combined PET/MRI scanner. Dr. Lee-Poprocki notes that calculations often were initially completed using the bulk assignment method, which has remained sufficient for certain applications, getting more sophisticated over time to provide additional detail, and later, through code-based assignments. As deep-learning algorithms evolved through training, they recently became the promising method for synthetic CT; however, the availability of large datasets remains a concern for achieving accuracy with the relatively new technology, Dr. Yang says.
“If we use AI technology to generate synthetic CT, we need a bigger and diverse database that includes MR and the corresponding CTs. If we want to include more patient data from different institutions, the problem is we’re all using different protocols and machines,” Dr. Yang explains, noting that these discrepancies affect data quality and, therefore, algorithm accuracy. “Ideally, we want bigger data and diverse data for our model training, but it is important to acknowledge that sharing data across different institutions may raise concerns related to patient privacy and policy. Additionally, the implementation of image standardization processing can assist in mitigating disparities in image quality resulting from variations in imaging protocols and scanners.”
Joshua Palmer, MD, clinical associate professor of radiation oncology at The Ohio State University Wexner Medical Center, says some radiation oncologists are hesitant about implementation of synthetic CT generated through MRIs using AI.
“If this deep-learning algorithm creates a CT scan and the density is incorrect—not the same as what the [standard] CT would have been—we don’t want to introduce an error that causes harm to the patient,” Dr. Palmer says. “This is why we’re purposely doing a prospective clinical trial to create evidence to support that it is safe.”
At Ohio State, Drs. Lee-Poprocki and Palmer are researching synthetic CTs from MR for brain metastasis radiosurgery patients who typically require an up-to-date MRI. The 30 participants undergo standard-of-care CT scans for treatment planning, which are later compared with synthetic CT from MR sequences from technology provided by Siemens Healthineers. To restrict movement and ascertain higher-quality images, patients are scanned wearing immobilization masks developed by QFix Systems.
“We will have the innate data of what the calculation shows in the CT sims. If we can demonstrate there’s very high agreement—that it’s essentially the same dosimetry on both the synthetic CT and real CT—in each of our patients, we show that it’s a high-fidelity workflow,” explains Dr. Palmer. “Then we have gotten over the hump to say, ‘Yes, we have the data to support that this technology is safe to roll out for patients.’”
Dr. Lee-Poprocki is also developing a model for performing phantom, or developing quality assurance (QA), tests on the scanning technique and treatment accuracy over time.
“Now that patients are wearing immobilization devices, we assume they’re pretty close in position and we want to make sure the planning qualities are clinically equivalent so the physicians feel comfortable,” Dr. Lee-Poprocki explains. “If we can also produce the same dose plans for radiation surgery, we can say [the synthetic CTs] are good to go.”
Patient Setup and Workflow Implications
To understand the inherent benefits of synthetic CT production, it’s important to review conventional workflows for treatment planning patients. Brain tumor patients, for example, will typically have an MRI within a few days up to 2 weeks before radiation therapy begins, as well as a CT exam in the treatment position to ascertain density information. The fusing of these images allows the treatment planning team to delineate the target and perform associated treatment planning functions.
Dr. Palmer notes that if the MRI is performed recently and within his Ohio State network, there is not often a need to perform an additional scan from which a synthetic CT can be produced. The treatment team will simply use the MRI on file and perform a standard CT simulation. However, when a patient comes from a referral site outside the network, the MRI is often not detailed enough for radiation therapy planning, occurring in about 25% of his patients, he says. The treatment planning team will perform a repeat MRI, producing the synthetic CT, which is faster and more convenient for the patient.
“If we’re repeating the scans, instead of duplicating efforts doing an MRI and a CT scan simulation, we just do the MRI simulation,” Dr. Palmer says. “It really facilitates outside patients coming in, saves them a trip, and shortens their workflow so they can immediately get treated. It’s smoother,” he says.
Dr. Yang adds that AI technology enables an accelerated clinical workflow of cancer radiation therapy through auto-contouring and auto-treatment planning. “If patients get a diagnostic MRI in radiology, they could potentially get treated that same day. It can save a lot on medical costs, and is also safer with no radiation, and better for the patient. If we let several weeks go by after the patient is diagnosed, that tumor can grow and grow. Integrating synthetic CT is helpful here.”
Typically in acquiring the images, radiation oncology coordinates with radiology regarding the table-specific needs. It’s optimal for the diagnostic MRI scanner to have a similar couch to the treatment machine and tabletop adaptors for the mask placement to enable better motion control. AI technologies have the potential to overcome certain limitations in MR imaging, such as extended scanning time, inadequate image resolution, artifacts, distortions, and inhomogeneity, Dr. Yang notes.
Future Requirements
For synthetic CT to gain traction in radiation therapy, clinical implementation will require improved QA, more comprehensive datasets for AI application, and enhanced collaboration among team members.
“Some more work needs to be done to develop a good quality assurance process. We need to make sure we’re providing high-quality care with minimal error in the MRI acquisition,” Dr. Lee-Poprocki says.
Achieving these advancements will help build trust among radiation oncologists in generated CT data, Dr. Yang says. “A lot of the time, physicians are excited, but others do not trust [synthetic CT]. I believe appropriate training and education would change their minds.”
Further, current AI models for synthetic CT typically apply to specific sites such as brain, head and neck, or pelvis. Generalized models are preferable for easier implementation, says Dr. Yang.
“We need bigger data to treat more generalized, robust AI models. With a larger database, hopefully all AI models will be generalized for all sites,” Dr. Yang says, adding that larger databases will require high-performance computational resources. As these advancements materialize, MRI-guided adaptive radiation therapy for photon and proton therapy will expand to support near-real-time guidance with MRI only.
Dr. Palmer concludes, “As we develop better AI technologies and deep learning algorithms, our ability to use MRI imaging and try to eliminate additional radiation to the patient, improve workflow, and increase satisfaction will grow. We’re very intentional in both ensuring outcomes are excellent while trying to help improve the patient experience. We hope to deliver this with MRI simulation technology.”